Keywords
Abstract
The development of novel small drug molecules is a complex and important cross-disciplinary task. In the early stages of development, chemoinformatics and bioinformatics methods are routinely used to reduce the cost of finding a lead compound. Among the tools of medicinal chemistry, docking and molecular dynamics occupy a special place. These methods are used to predict the possible mechanism of binding of a potential ligand to a protein target. However, in order to perform a docking study, it is necessary to know the spatial structure of the protein under investigation. Although databases of crystallographic structures are available, the three-dimensional representations of many protein molecules have not been reported. There is therefore a need to model such three-dimensional conformations. Several computer algorithms have been published to solve this problem. AlphaFold is considered by the scientific community to be the most effective approach to predicting the three-dimensional structure of proteins. However, the scope of its application in medicinal chemistry, especially for virtual screening, remains unclear. This review describes methods for predicting the three-dimensional structure of a protein and provides representative examples of the use of AlphaFold for the design and rational selection of potential ligands. Special attention is given to publications presenting the results of experimental validation of the approach. On the basis of performed analysis, the main problems in the field and possible ways to solve them are formulated.
The bibliography includes 154 references.
1. Introduction
The development of new small drug molecules is a high-tech, phased, and expensive process[1]. To reduce the time and financial expenses in the early stages of development, various computational modelling methods[2, 3] are typically employed. This allows for the analysis of, for example, the probable mechanism of ligand binding to a target, selection of the most promising molecules for high-throughput virtual screening (VS)[4] stage, prediction of their pharmacokinetic parameters[5], suggestion of retrosynthetic pathways[6], and evaluation of other important properties. Historically, the following key approaches to virtual screening have formed[7]:
— ligand-based drug design, LBDD;
— structure-based drug design, SBDD.
Both approaches have their strengths and weaknesses; however, the latter method can be considered more accurate and informative. It is evident that to carry out typical SBDD (structure-based drug design) procedures, one must have access to the three-dimensional structure of the target (in most cases, this is a protein molecule; see Section 7 for the full names of the proteins reviewed in this overview). Such information can be found in specialized sources, such as the PDB a database, which contains data on the three-dimensional structures of proteins[8]. However, for many targets, especially new ones, such information may be lacking. In these cases, the method of constructing three-dimensional structures by homology is often employed[9] if crystallographic data of comparatively high resolution (preferably not more than 3 Å) are available for a closely related template. It should be noted that until recently, there were no available effective and accurate methods for solving such a task within the scope of VS.
The rapid development of machine learning and artificial intelligence methods has led to the creation of computational algorithms that, among other things, allow for the prediction of three-dimensional protein structures[10-12]. This undoubtedly holds great significance for bioinformatics, structural biology, enzymology, in particular for the study of enzyme action mechanisms and self-regulation[13], the investigation of oncogenic mutations[14], modelling of protein–protein interactions[15], etc. Such methods play an important role in X-ray crystallography[16, 17] and in conducting broad statistical studies of various functional and structural properties. Overall, information about their threedimensional geometry is necessary for the creation of protein molecules; this information also contributes to a deeper understanding of protein functions under normal physiological conditions and in pathologies.
Despite the obvious successes in predicting the threedimensional structure of proteins, the effectiveness of such an approach depends on the specific task. In medicinal chemistry, significant attention is paid to the mechanism of interaction between the ligand (small molecule) and the binding site through the formation of chemical bonds between the atoms of the ligand and the amino acids that constitute the binding site, which largely determines its affinity and selectivity. Furthermore, it is necessary to consider the conformational flexibility and heterogeneity of the sites[18], the nature of the solvent molecules[19, 20] filling the site cavity, including bridge water molecules, and in some cases, the critical role of just one or two amino acids that determine the activity and selectivity among the closest homologues in the family, for example, as in the case of CDK5/2 kinases (Asn144/Asp144)[21], Wee1/2 (Asp386/ Ala386)[22]. The solvent effect for polar amino acids forming the active site of the enzyme is generally weaker. The spatial position of such ensembles is deterministic, which is reflected in their B-factor values. The relatively low conformational mobility of amino acids is ensured, among other things, by hydrogen bonds. However, differences in substrate specificity are observed even among close homologues, despite their considerable overall spatial similarity. For example, among ATP-competitive kinase inhibitors (ATP stands for adenosine triphosphate), selectivity is determined by interaction both with amino acids in the hinge region and with distant pockets, which, in particular, defines the type of kinase inhibitors[23]. In other words, in many protein molecules, depending on their functions, there are conservative areas the spatial geometry and mutual arrangement of which can be predicted with relatively high accuracy by modern methods. Examples include evolutionarily developed domain structures and regions characterized by relatively high mobility, which often influences the binding of a ligand to the protein target (protein–ligand docking).
Molecular docking methods play an important role in the arsenal of the modern medicinal chemist. They are used to assess the possibility of ligand binding to a specific area in the target structure. It is clear that in modelling scenarios where the amino acids lining the binding site remain stationary and the three-dimensional geometry of the ligand is optimized to minimize the scoring function, even seemingly minor differences in the pocket geometry can lead to invalid results from the computational experiment. When using flexible docking, which optimizes the positions of the ligand atoms and surrounding amino acids simultaneously, the effect of such differences is mitigated, but assessing the result remains challenging, as in most cases only one ‘trajectory’ along the gradient of potential energy is selected. A more detailed mechanism of ligand interaction with the target can be obtained through a dynamic description of the binding process using molecular dynamics (MD) methods[24, 25]. However, such approaches involve significant time expenditures and require substantial computational power. It should be noted that not all ligands bind according to the key–lock principle; many interact with the binding site according to the induced fit mechanism, which is associated with conformational transitions in the pocket structure. Similarly, drugs acting on allosteric sites lead to changes in the geometry of the binding site with the endogenous ligand, unless the discussion involves the regulation of protein– protein interactions. Given the above, the question of the applicability limits of neural network algorithms, which predict the three-dimensional structure of proteins for the needs of medicinal chemists, especially in the early stages of development, remains open.
2. Computer algorithms for predicting the three-dimensional structure of protein molecules
Anfinsen’s seminal study[26] conducted in the 1970s demonstrated that the tertiary structure of a protein depends on its amino acid composition. The development of sequencing methods has allowed for the identification of more than 2.6 billion nucleotide sequences as of 2021, of which more than 200 million have been translated into corresponding amino acid sequences. However, the amino acid composition alone provides only a limited understanding of the biological functions of a protein, since these functions are determined by its spatial structure. For instance, nearly 30% of the human proteome consists of disordered structures that perform their functions despite the lack of well-defined tertiary structures.[27] Specifically, the active conformation of such proteins may form at the moment of interaction with a partner molecule[28]. Owing to crystallography and NMR spectroscopy methods, there are currently more than 200,000 three-dimensional conformations of protein molecules in the protein structure database, which is less than 0.1% of the total number of sequences in the UniProt database. Over time, the gap between the number of known protein sequences and experimentally determined tertiary structures is narrowing. In recent years, the spatial structures of many proteins have been modelled using various computational methods. The development of high-precision protein structure prediction methodologies is the most promising approach to bridging the discrepancy between the number of known amino acid sequences and the number of experimentally determined three-dimensional protein conformations.
Research aimed at predicting the tertiary structure of protein molecules began in the mid-20th century when the first Ramachandran plots[29] were published and methods for constructing three-dimensional structures based on amino acid sequence homology were proposed[30, 31]. Later, the first software emerged, including the MODELLER and SWISS-MODEL algorithms to model the protein tertiary structures based on homology. For instance, for proteins with amino acid homology over 50% relative to the template, the average root mean square deviation (RMSD) may not exceed 1 Å compared to the experimentally determined structure. Alignment is typically carried out using the Needleman–Wunsch or Smith–Waterman algorithm[32, 33], with relatively fast alignment achievable using programs like BLAST[34], PSSMs and HMMs[35, 36], 3D-Jury[37] and LOMETS[38]. It is clear that as homology decreases, the deviation increases, averaging 2–5 Å for homology around 30–50%, while for proteins with <30% homology, modelling results are mostly considered unreliable[39, 40]. Identifying remotely homologous templates is no less challenging[41], reducing the likelihood of selecting the most suitable structure for modelling.
Currently, various neural network algorithms for the modelling of three-dimensional structures of protein molecules are available. The general goal of such approaches is to predict the spatial position of each atom in the protein molecule based on its amino acid sequence. Some methods use available templates for modelling, while others do not require the presence of homologous three-dimensional structures. During the scientific competition CASP-14 (CASP is Critical Assessment of Protein Structure Prediction), it was shown that using an endto-end approach based on deep learning algorithms[42], it is possible to predict the coordinates of amino acid atoms in singledomain protein molecules with high accuracy, in particular without the direct use of templates. The quality of the prediction was weakly correlated with the number of available homologues. The most well-known algorithms that allow modelling of the tertiary structure of a protein based on its amino acid sequence are presented in Table 1. As an example, let us take a closer look at some of them.
One of the successful strategies for modelling the threedimensional structures of protein molecules with distant homology is I-TASSER[56]. For template searching, the algorithm uses the threading method, performed by the LOMETS (local meta-threading server) 38 module. In the structure of the identified templates, closely related fragments and their threedimensional conformations corresponding to sections of the studied amino acid sequence are determined. These fragments are then assembled into a set of tertiary conformations of a ‘virtual’ protein, which has the maximum similarity in relation to the studied amino acid sequence. This stage is carried out using the Monte Carlo method and the DOOP (docking decoybased optimized potential) algorithm[57], which uses empirically distance-dependent interactions between pairs of atoms and considers elements of the secondary structure. As a result, thousands of possible three-dimensional structures are generated. Next, a clustering procedure based on structural similarity is carried out. Statistical processing of the clustering results allows for the identification of the most probable three-dimensional structures from the formed clusters, with the scoring function being calculated using SPICKER[58]. Then, a reassembly procedure is carried out using the LOMETS, TM-align (Ref. [59]), and IRP (inherent reduced potential) algorithms. The final fullatom three-dimensional protein model is created during optimization by the modified REMO H-Bond algorithm[60] using a set of empirical rules. Unaligned sections are also minimized. A key feature of I-TASSER is the use of sets of structural templates. Examples of using this algorithm to solve various tasks in the field of bioinformatics and the development of new drug molecules are described in a number of publications (see, for example, Refs [61-65]).
Before the emergence of AlphaFold, the ROSETTA algorithm[44, 66] was leading in the CASP (Critical Assessment of Protein Structure Prediction) competitions. The program is based on the fragment insertion approach, which uses relatively short segments from known protein structures to initiate modelling. For each small fragment (3–9 amino acids) of the protein sequence, the algorithm searches for suitable threedimensional templates and randomly selects them as starting representations with a homology threshold of 50%. Alignment is performed using the PSIBLAST algorithm[67], involving full pairwise comparison. Fragments are described by torsion angles from a library of training examples (crystallography data with a resolution of no more than 2.5 Å). For predicting secondary structures, ROSETTA uses methods like Psipred, SAM-T99, and JUFO; torsion angles are compared with corresponding angles from the training examples, and erroneous results are excluded from consideration. Next, assembly and optimization procedures of the initial three-dimensional conformations are carried out using the Monte Carlo method and an energy function. At this stage, the algorithm introduces random changes to the values of torsion angles and ranks changes with a certain probability, according to the Metropolis criterion[68]. ROSETTA uses a specialized empirical function for calculating potential energy to evaluate the results, taking into account the LennardJones potential, solvation, and intermolecular hydrogen bonds. The goal of the optimization stage is to select the most stable conformations for a given amino acid sequence. A feature of the program is that the algorithm does not just create one structure and evaluate it but generates multiple possible variants and ranks them based on the scoring function. In each iteration, ROSETTA refines and improves the prediction outcome.
The I-TASSER and ROSETTA approaches are generally similar; however, the latter does not use the threading procedure and employs its own empirical function to estimate potential energy using the Metropolis criterion. ROSETTA uses a multitude of different starting structure variants, and the databases of initial templates also differ. The program operates in multiple threads, resulting in a large set of structures, with close conformations being clustered together. Sparse groups are not considered, and from the remaining ones, those with the lowest average energy are selected. In the first stage, ROSETTA essentially annotates the studied amino acid sequence for the potential presence of conservative secondary conformations, comparing small fragments of the sample with a reference database. This procedure is probabilistic in nature, as it involves many independent runs. Each amino acid is represented by a limited set of anchor points (practically, harmacophore centres) to accelerate the modelling process in the early stages. As a result, the program presents several variants of possible packings with minimal potential energy values. A full-atom model is then constructed and undergoes final optimization. However, the program does not perform best with proteins longer than 150 amino acids and requires substantial computational power and typically significant time expenditures. Examples of using ROSETTA algorithm can be found in a number of publications[69-72].
SPARKS-X is the latest version of the program for sequence, secondary structure profiles, and residue-level knowledge-based energy score, which predicts the three-dimensional structure of a protein based on its amino acid sequence (previous versions include SPARKS, SP2, SP3, SP4, and SP5)[36]. The algorithm is based on the method of multiple sequence alignment[67], secondary structure prediction, its own scoring function[73] (modified SKSP method)[74], comparison method using template structures[75], evaluation of the solvent accessible surface area (SASA)[76], and a torsion angle prediction module[77] using machine learning methods. In the CASP-6 and CASP-7 competitions, the SPARKS, SP3, and SP4 programs were among those that showed the best results[78, 79]. Unlike previous versions, SPARKS-X integrates an updated energy function using hidden Markov models[80]. This improvement has enhanced the prediction quality for secondary structure based on the primary sequence: Q3 = 81–82% (the proportion of correctly predicted substructures; Q3 is a calculated parameter reflecting the accuracy of predicting a protein secondary structure: α helices, β-sheets, and loops[81]). The mean absolute error values for \( \phi \) and \( \psi \) angles were 33° and 22°, respectively, with a determination coefficient R2 = 0.74 for SASA. The alignment procedure on template sequences is implemented using a modified function from SP5 and the PSIBLAST method, with the optimization of the scoring function being performed according to the Smith–Waterman algorithm[33], and template structures ranked according to the value of the standard statistical Z-score. Specifically, the developers managed to improve the overlay of Cα atoms according to the obtained values of the scoring function MaxSub (Ref. [82]) for different protein families.
Protein secondary structure prediction involves several stages. First, a PSSM (position specific scoring matrix) is constructed using the PSIBLAST mutational profile and seven parameters reflecting steric hindrance, hydrophobicity, volume, polarizability, isoelectric point, and the probability of forming α-helices and β-sheets[83]. These parameters, along with the PSSM, serve as the input vector to a neural network. The secondary structure is evaluated using the composite SKSP7 scoring function[84]. In the next step, another neural network with a RealSPINE architecture[85] predicts the SASA (solvent accessible surface area) for amino acid residues, using the PSSM, the above parameters, and the predicted secondary structure as input vectors. Then, the SASA values, secondary structure, PSSM, and parameters are used to predict torsion angles (\( \tau_0 \)). Subsequently, the secondary structure for helical segments and β-sheets, to which incorrect torsion angles were initially assigned, is predicted using SASA, \( \tau_0 \) values obtained in the second stage, PSSM, and parameters (refinement procedure). The newly obtained secondary structures, along with SASA, PSSM, and the specified parameters, are used to predict new torsion angles (\( \tau_1 \)). In the final stage, a neural network is used, the predictive ability of which was assessed using examples from the DSSP database. The authors chose PSSM, parameters, SASA, and \( \tau_1 \) as the feature input vector. Neural networks were trained using a reference set of structures consisting of 2640 protein molecules obtained from the PISCES database[86] with homology not exceeding 25% (crystal resolution less than 3 Å), excluding molecules with a chain length exceeding 500 residues. Using test examples from CASP-9, it was demonstrated that the SPARKS-X algorithm can model three-dimensional structures of protein molecules, with the quality of modelling being comparable to that of ROSETTA. Results of the SPARKS-X algorithm can be found in a number of publications (see, for example, Refs [87-89]).
3. AlphaFold algorithm
The AlphaFold algorithm has recently gained widespread recognition and attracted the attention of many specialists in the field of structural biology and bioinformatics. In 2023, the developers of this program, D.Hassabis and J.Jumper, were awarded the Lasker Award in the category of Basic Medical Research. The AlphaFold program, built on artificial intelligence, was trained on a large number of examples. Currently, two versions of the program are available. In December 2018, AlphaFold-1 ranked first in the overall CASP-13 competition. The program successfully tackled the task of predicting the spatial structures of proteins for which no template structures were available, despite partially similar amino acid sequences. In 2022, AlphaFold-2 won the CASP-14 competition: the program was able to relatively accurately model the threedimensional structure of 35% of proteins for which close templates were absent, and for 77% of proteins with available templates[52]. The new version demonstrated higher accuracy compared to other similar algorithms and scored more than 90 out of 100 possible points for two-thirds of the proteins in the GDT (global distance test), which is used to assess how accurately a three-dimensional protein structure has been predicted compared to experimental data. For 88 out of 97 proteins, the AlphaFold-2 algorithm showed better results than other methods. In 2021, an article[52] was published (with more than 15000 citations as of October 2023) describing the AlphaFold-2 algorithm along with open-source software and the corresponding database.
In the first stage of the AlphaFold-1 algorithm, the sequence of the protein of interest is aligned with the sequences of other proteins with known three-dimensional structures (multiple alignment). Next, using the coevolution matrix, pairs of amino acids forming key (conservative) interactions are analyzed. A high correlation corresponds to critical contacts and indicates that the amino acids are close to each other in three-dimensional space. In homologous proteins, such pairs remain unchanged or change synchronously. Unlike other programs, AlphaFold-1 predicts pairwise distances between Cβ atoms, providing discrete probability distribution densities for each amino acid in the form of matrices. A distance not exceeding 8 Å is used as the interaction threshold. The three-dimensional structure is reconstructed using a potential (analogous to a potential energy scoring function) dependent on the dihedral angles \( \psi \) and \( \phi \), pairwise positioning of Cβ atoms, and volume overlaps. Optimization of this function is achieved through gradient descent. The locations of Cα atoms are mechanistically determined from known C–Cα–N distances. Modelling is performed using a ResNet class convolutional neural network[90] with a training sample of over 30000 crystallographic structures. A key feature of AlphaFold is the structure of its input and output data.
Unlike AlphaFold-1, the end-to-end model AlphaFold-2 uses a transformer architecture with an ‘attention mechanism’. Training was conducted on 170000 examples, which provided more accurate predictions in the CASP-14 framework and sped up the algorithm’s operation. For searching the closest homologues and aligning amino acid sequences, the program uses the HMMER method (hidden Markov models)[91], based on Markov models (chains), and databases such as Uniprot and MGnify, while the HH-suite method[92] is used to search for the closest three-dimensional structures in the PDB database. Like AlphaFold-1, the new version operates with matrices of pairwise distances, but in the absence of a suitable template, it is filled with default values. The neural network receives a lowdimensional continuous vector representation of the alignment and pairwise correspondence (vector representation) as input and iteratively improves their quality on deeper layers. As a result, the model returns the predicted three-dimensional structure of the protein.
AlphaFold-2 essentially consists of three modules: Evoformer (vector representation, computational block with trainable weights), the structural module (generation of three-dimensional structure), and OpenMM (optimization of atom coordinates–system relaxation). The main neural network of AlphaFold-2 predicts the positioning of the peptide backbone, while Deep ResNet outputs dihedral angles that describe the positions of amino acid side chains. The Noisy Student Training approach (semi-supervised learning) is used for training[93]. In the initial stages of the system operation, all elements of the peptide backbone are placed at the origin, and then the coordinates are updated by the structural module, taking into account the information received from the IPA (invariant point attention) function. AlphaFold-2 uses the FAPE (frame aligned point error) as its loss function, which is independent of changes in the global coordinate system. In terms of operation and data processing logic, AlphaFold-2 is virtually indistinguishable from the first version, but the neural network architecture and the training algorithm itself have been significantly modified. This, in particular, considering the use of a large volume of training data and the coevolution matrix, has allowed AlphaFold-2 to take a leading position in the field[94].
We did not intend to conduct a detailed comparison of algorithms and their neural network architectures for predicting the three-dimensional structure of protein molecules. A recent publication[95] presents the results of a comparative analysis of the AlphaFold-2 and RoseTTAFold algorithms and describes details of their architecture. For instance, it highlights the significantly higher efficiency of the two-track neural network architecture used in AlphaFold-2 compared to RoseTTAFold. Furthermore, as another distinguishing feature, the authors pointed out that the multiple alignment parameters are updated in AlphaFold based on pairwise characteristics through the direct attention mechanism, which provides a more accurate prediction of the spatial positioning of atoms. AlphaFold-2 employs end-to-end training, updating all model parameters through the backpropagation of errors from the loss function, which is calculated from the three-dimensional coordinates after passing through several SE(3)-equivariant layers of the transformer neural network. More details on the algorithm features can be found in the supplementary materials to the mentioned article.
Although there are quite a few publications to date with examples of using the AlphaFold-2 algorithm for solving bioinformatics tasks[96], its application for the development of new small drug molecules, especially at the early stages, remains unexplored. To be successful, a modern medicinal chemist must have deep knowledge in areas such as organic synthesis, classical medicinal chemistry, and chemoinformatics. It was mentioned earlier that methods of molecular docking or MD (molecular dynamics) are often used to predict and understand the mechanisms of molecule binding to a chosen target, based on which a specialist can evaluate the potential of a small molecule and modify it to improve the affinity. This requires the three-dimensional structure of the protein molecule. Problematic are those cases when it comes to a protein for which no data on the three-dimensional structure are available and there are no closely related analogues. Considering that the researcher’s primary focus is precisely on the area of potential binding of a small molecule to the target, the question of how accurately the AlphaFold algorithm can predict the geometry of the binding site adapted for docking modelling remains open. Below, recent scientific publications are detailed, where the AlphaFold algorithm was applied with the aim of developing drug molecules and virtual screening.
4. AlphaFold exploitation for small drug molecule development
4.1. Virtual screening Comparing the results of docking
Comparing the results of docking simulations obtained using the Glide module (see d and Ref. [97]) with data from the DUD-E database (see e and Ref. [98]) allowed for a preliminary assessment of the rationality and efficiency of using model structures available in AlphaFold for virtual screening (VS) in comparison with the use of crystallographic data. Within the scientific community, the DUD-E database, despite its shortcomings, is considered to be a standard set for conducting independent testing of computer models[99]. As the subject of their study, Zhang et al. [98] selected holo (in complex with a ligand) and apo (without a ligand) forms of proteins corresponding to the AlphaFold model and their optimized variants, which were obtained using the induced fit docking molecular dynamics (IFD-MD) protocol.f Two different forms (holo and apo) were chosen based on the premise that docking simulation results depend on the geometry and composition of the binding site, which in many cases differ for the mentioned forms.
IFD-MD is a separate module that combines traditional approaches and docking functions, pharmacophore analysis of the binding site, solvation assessment (WScore function), sampling of amino acid side chains, and partially MD (molecular dynamics) methods to optimize the ligand position (modelling the induced fit mechanism) in its algorithm. The algorithm, which requires the presence of a template structure of the ligand–protein complex, was used, in particular, to obtain a greater number of holo-like structures based on apo-forms of AlphaFold protein models. It was noted that the MOE programg also implements similar functionality in the forced alignment mode. The authors [98] pointed out that their approach is applicable exclusively to protein models the binding site of which has the peptide backbone correctly positioned. The original AlphaFold models were compared with modified and optimized variants and with relevant experimental data. It was noted that docking in AlphaFold models for which experimental data on the threedimensional structure of protein molecules are not available does not guarantee the discovery of hit molecules. It was shown that the application of the IFD-MD method leads to a significant improvement in docking results according to the BEDROC enrichment coefficient.h However, the cited publication[98] clarifies that all examples from the DUD-E database were already available in the PDB at the time of training the AlphaFold algorithm.
The DUD-E database contains structural information on 102 protein molecules belonging to various families, including kinases, nuclear receptors, proteases, and others. For each class of targets, a set of active ligands and inactive structurally similar molecules is available. For analysis, a set of 40 proteins was used, for which both forms (holo and apo) are presented in the database, by analogy with the study by Im et al[100]. Structures without analogues among the AlphaFold models for which only covalent ligands were indicated as targets were excluded from this set. After the filtering procedure, 27 proteins remained. Since for a number of objects, such as BRAF (B-Raf protooncogene), EGFR (epidermal growth factor receptor), IGF1R (insulin like growth factor 1 receptor), ITAL (integrin α-L), and RXRA (retinoic acid receptor-А), the binding sites of AlphaFold-2 (AF2) are blocked by an excess of amino acids, an alignment of the primary sequence between the AF2 model and the holo-form was performed, and then peptide segments before the first and after the last amino acid in the sequence corresponding to the holo-form were removed.
These operations resulted in a sample of modified AlphaFold models more suitable for reproducing the docking. Then, for all proteins and complexes, a preprocessing procedure was conducted using the ProteinPreparation module, during which hydrogen atoms were added, missing peptide chains were built, and protonation was carried out in accordance with the PROPKA (protein pKa) algorithm at pH 7.4[101], considering functionally significant tautomeric forms of histidine. Next, hydrogen bonds were optimized and a potential energy minimization procedure for the entire protein molecule was conducted. Active and inactive ligands from the DUD-E database underwent a standard preparation stage using the LigPrep module, with a maximum of 32 permissible starting conformations (default value). Cofactors and coenzymes that play a significant role in ligand binding and/ or structural stabilization of the binding site were positioned in the corresponding AlphaFold models based on alignment, and their positions were refined during minimization. Using the IFD-MD protocol, models of complexes (no more than 5 different poses for each ligand) were obtained and used to perform docking simulation of active and inactive molecular structures. In some cases, the authors 98 used the PLDB module to select other reference molecules from the PDB database based on their structural similarity (Tanimoto metric, RDKit fingerprints) to the molecules associated with a specific target from the DUD-E set. Based on the results of molecular docking (Glide module, standard SP protocol, Table 1), one variant with the best scoring function was selected for each example. To compare the geometry of the binding sites (amino acids within no more than 5 Å from the ligand atoms were considered when calculating RMSD values) for holo- and apo-forms, as well as original and modified AlphaFold structures, the SiteMap module was used.
As a result, it was shown that the spatial geometry of the binding sites in AlphaFold structures resembles more closely the holo-forms (average RMSDbackbones were 1.48 and 1.17 Å, and RMSDside chains were 2.15 and 1.83 Å for apo- and holo-forms, respectively). From the data presented in Table 2, it is evident that when using modified models, the docking results are comparable with those for the apo-forms of proteins but are significantly inferior to the results for holo-forms, which is explained by the principle of induced fit and protein-specific features, examples of which can include the DFG-in/out and αC helix in/out conformations of the kinase binding site. The applicability of such models for the needs of standard virtual screening is quite limited. Comparative target-specific data for the same sample but with the addition of IFD-MD-optimized structures are presented in Figure 1.
Based on the results, it can be concluded that holo- and apo-forms with low RMSD values in the binding site area relative to the holo-forms yield the best docking results. A similar but less pronounced trend is observed for AlphaFold models. For example, in the RXRA protein molecule, the C-terminal helical segment is located inside the binding site, significantly affecting the docking results. Zhang et al. [98] noted that in the case of modified AlphaFold models, incorrect ligand positioning results were due in part to incorrectly defined rotamers of amino acids lining the site. Docking results for holo-and apo-forms, as well as for modified AlphaFold models compared to their IFD-MD optimized variants, in which reference ligands were preliminarily placed after the alignment or docking procedure, are presented in Table 3. However, it was emphasized that the poses obtained during docking did not always correspond to the true ones. IFD-MD-optimized models showed results comparable to those for holo-forms (see Table 3), especially in the cases where the entire set of docking models was used (see above). The authors effectively demonstrated the efficiency of using the IFD-MD method in relation to the EGFR kinase, for which holo- and apo-forms, as well as the modified AlphaFold model, were found to be unsuitable for docking construction. In particular, during optimization, different rotamers were obtained for the amino acid residues Met766 in the C-loop and Cys775, freeing additional volume in the site for ligand binding, while the Glu762 side chain was displaced from the site. Conformational changes occurred to Phe856 in the DFG segment [Asp(D)–Phe(F)–Gly(G), a conservative segment in the structure of kinase pockets] and Thr790 (the gatekeeper region). Together, these spatial changes allowed the overall geometry of the binding site to be approximated to the holo-form and, consequently, improved the docking results. Similar experiments were conducted using reference structures of active molecules with low structural similarity to the ligands used previously, improving docking results, although this experiment involved significant time and computational expenses. Additionally, an approach was proposed that considers the influence of cofactors and coenzymes.
Based on the experiments, Zhang et al. [98] concluded that unmodified AlphaFold structures yield results in standard docking modelling similar to those for apo-forms, which are noticeably inferior to the results for holo-variants. However, the use of the IFD-MD method significantly improves the quality of prediction. The authors did not conduct virtual screening using this algorithm to search for potential ligands, which does not fully assess the capabilities of the described approach, particularly in the field of developing new hit molecules acting on targets that do not have close homologues among available experimental complexes.
Weng et al. [103] used the AlphaFold model built for the protein WSB1 (WD repeat and SOCS box-containing protein-1) within the framework of virtual screening (VS) to search for inhibitors of its activity. This protein consists of seven WD40 domains (N-terminus) and one SOCS box (C-terminus). It promotes the growth and development of tumour cells by blocking the activity of the tumour suppressor protein pVHL and activating the transcription of the HIF-1α gene. The conducted virtual screening identified a number of compounds as potential ligands to the said protein, among which G490-0341 (1), G610-0188 (2), Y043-6168 (3), and Y044-5019 (4) showed the best results in subsequent MD studies (Figure 2). Programs AutoDock-GPU (Ref. [104]) and GlideSP (Schrödinger, Maestroi ) were used to model the docking of structures.
In the first stage, the AlphaFold model was preprocessed using the MOE program, QuickPrep module, where structural correction, addition of hydrogen atoms, removal of unbound water molecules, and energy minimization were performed, while in the Maestro program, ligand structure preparation was carried out using the LigPrep module. The peptide ligand D2 (type 2 iodothyronine deiodinase) was used to identify the binding site using MD [binding pose meta dynamics (BPMD) method was applied]. The initial docking modelling was performed using the AutoDock-GPU program, with molecules from the ChemDiv collectionj serving as the subjects of study. Based on the experiment results, more than 127000 molecules with a scoring function not exceeding –10 kcal mol–1 were selected, which were then examined in a stepwise docking using the Maestro program. During the experiment, a maximum of 20 possible poses with a Glide XP score £ –8.0 kcal mol–1 were obtained for each structure. Based on the modelling results, 20 most promising structures were selected, for which the BPMD method was also applied to refine their poses. It was shown that compound 1 is capable of forming strong bonds with key residues Asp175 and Arg315, forming a stable complex. The authors [103] did not present the results of biological testing of the selected molecules, which does not fully assess the contribution of the AlphaFold model to the results of the described experiment.
Wong et al. [105] used AlphaFold structures and docking modelling to predict the possible interaction between 296 E. coli proteins and 218 antibacterial molecules. The approach was validated for 12 proteins in vitro. The study results showed an average auROC value of 0.48, indicating low efficiency of the approach. Reassessment of docking poses using machine learning methods improved the model characteristics (auROC = 0.63–0.71). The quality of prediction varied depending on the used activity threshold and averaged 41–73%. A negative sample of 100 inactive molecules was used. In the first stage, the authors investigated the antibacterial activity (inhibition of E. coli K-12 BW25113 growth) for a library consisting of more than 39000 molecules, which included known antibiotics as well as natural compounds and synthetic molecules with high structural diversity (molecular weight from 40 to 4200 Da). Molecules were tested at a relatively high concentration (50 mM). Compounds that showed activity of at least 80% (218 molecules) were selected as hits, about 80% of which belonged to known classes of antibacterial agents, including β-lactams, aminoglycosides, tetracyclines, fluoroquinolones, etc. The docking procedure for the selected structures was carried out using AutoDockVina. As an independent in silico control, complexes available in the PDB database were used.
As a result, over 64000 poses for active molecules and over 29,000 for inactive ones were predicted. At threshold scoring function values of –7 and –5 kcal mol–1, respectively, 9.6 and 31% of molecules were classified as active against at least three proteins, while out of 296 proteins, 178 and 216 proteins showed a likelihood of binding to at least three active molecules. For inactive compounds, these indicators were respectively 86 and 99 molecules, and 137 and 204 proteins. Based on these results, it can be concluded that the model has a low classifying ability. In addition, the authors 105 assessed the predictive capabilities of the model using 142 antibiotic–target pairs described in the literature to replicate their binding mechanisms. However, unsatisfactory results were obtained in this case as well, regardless of the scoring function threshold. The quality of the model was studied based on in vitro testing results conducted for 12 E. coli proteins, including DNA gyrase, primase, helicase, NAD+-dependent ligase, polymerase, guanylate kinase, mur family proteins, and others, for which test systems were available that allowed for the direct assessment of molecule binding to these proteins and their activity (Figure 3).
In the biological experiment, it was found that 94 and 85 compounds at a concentration of 100 mM showed inhibitory capability exceeding 50% against murA and DNA helicase, respectively. Conversely, the number of molecules acting on DNA ligase and murC was much lower, at 4 and 5, respectively. For all primary hit molecules, IC50 (half-maximal inhibitory concentration) values were experimentally determined. It turned out that 45 molecules showed nonspecific activity and could be classified as PAINS (pan-assay interference compounds). The proportions of correctly predicted hits were 59 and 30% with scoring function thresholds of –5 and –7 kcal/mol, respectively (Figure 4). For false-positive molecules, this indicator was 66 and 22% respectively (Figure 4b). These results indicate that the model efficiency was not significantly different from the efficiency of a model with randomly selected molecules for screening. The auROC value for the 12 proteins ranged from 0.18 (murC) to 0.71 (gyrAB), with an average value not exceeding 0.5.
Similar results were obtained using auPRC (pose/ranking consensus). The authors[105] noted, in particular, that they observed no correlation between auROC (or auPRC) and pLDDT (predicted local distance difference test)[52]. A similar computational experiment was conducted using molecules of the same compounds the structures of which are available in the PDB database. However, no improvements in the prediction quality were established. Consequently, Wong et al. [105] used another docking modelling method, namely DOCK6.9 (Ref. [106]) and evaluated the influence of several scoring functions based on machine learning models (Figure 4c,d): RF-Score[107], RF-Score-VS[108], PLEC score[109], and NNScore[110]. The results showed that the RF-Score, RF-Score-VS, and NNScore functions, applied to docking modelling results in AutoDockVina, significantly improved the average auROC values: 0.62, 0.63, and 0.58 respectively (across 12 proteins). Among the main conclusions, it was noted that AlphaFold-2 models, having several drawbacks, including the inability to distinguish between active and inactive protein conformations[111], in conjunction with docking modelling results (ligand is mobile, protein atoms are not) in a high-throughput virtual screening mode, do not provide a prediction quality sufficient for rational selection of molecules for biological testing.
Scardino et al. [112] investigated the efficiency of highthroughput docking (HTD) using AlphaFold models and corresponding crystallographic structures of 22 proteins. The analysis provided the conclusion that the original AlphaFold models used for this purpose were of low efficiency. In particular, the authors noted the important role of proper model preparation before the virtual screening (VS) stage. In the direct experiment, both AlphaFold models and crystals from the PDB database were not modified. Standard docking procedures used programs such as AutoDock-4, ICM, rDock, and PLANTS, which differ in algorithms and scoring functions. The efficiency of docking in the model was assessed using ECR (exponential consensus ranking) and PRC (pose/ranking consensus) approaches[113, 114]. Before starting the experiment, the authors compared the AlphaFold models and their crystallographic analogues using pLDDT and three different RMSD values. For some models, the binding site was blocked by other parts of the protein, hindering correct docking. Nuclear receptors, for example, ESR1, ANDR, and PRGR, can exist in various conformational states, but in AlphaFold models, predominantly only one conformation corresponding to the agonist-bound state is realized. For such cases, correct PDB database analogues for comparison were chosen. In the AlphaFold docking, the ICM program showed the best results, but overall, the prediction quality was low [EF1-ECR = 8.8 and EF-PRC = 8.9, HRavg = 0.16; EF1-ECR is average enrichment factor at 1% (EF1) by exponential consensus ranking; EF-PRC is average enrichment factor by pose/ranking consensus; HR is hit rate]. Many targets had EF<3. For particular examples, the EF value was close to zero, with an average RMSD for ligand positions compared to docking results being 4.64 (in the case of cyclooxygenase-1, the RMSD was >10).
The docking results for ligands with AlphaFold models compared to crystals from the PDB database are presented in Table 4. It is evident that AlphaFold models are inferior to actual crystals, and this trend is characteristic of all four programs used for modelling. Comparable results were obtained for targets PRGR, PTN1, DRD3, and KITH, while for UROK, KPCB, ANDR, FABP4, ADRB2, and PYRD, modelling using PDB data showed better classifying ability, reflected in higher EF1-ECR values. The RMSD value for template ligands in the case of actual crystals, unlike AlphaFold models, was significantly lower (RMSDavg = 1.25). As in other publications, it was noted that the quality of the peptide backbone positions for the selected proteins is generally comparable to experimental data. This is not the case for the positions of side chains, especially in the binding pocket area, which undoubtedly affects the quality of the prediction in the context of virtual docking.
Based on the conducted experiment, the authors[112] concluded that the original AlphaFold models are mostly unsuitable for typical docking procedures, and this correlates with the observations of other researchers[98, 115], but preprocessing of such structures generally improves the prediction quality. In particular, the crucial role of water at the ligand-binding interface was demonstrated using HSP90 as an example: the modelled solvent molecule positions allowed for an almost 8-fold improvement in RMSD between the true position and the docking result (RMSD values were 0.8 and 6.3 Å, respectively, in the presence and absence of water).
Hekkelman et al. [116] described the AlphaFill method for the automatic preprocessing of AlphaFold models, specifically aimed at improving the quality of docking. The authors noted that AlphaFold models are not designed to predict the positions of molecules that do not structurally relate to the peptide backbone and amino acid side chains. For example, haemoglobin should be considered in a complex with heme, zinc fingers should be considered with zinc atoms; these cofactors or coenzymes provide many DNA-binding proteins with stability and functionally significant spatial organization. Metalloproteinases should be analyzed with metal atoms in the active site, which dictates their catalytic activity. Similar conclusions can be made for other classes of protein molecules, for example, kinases, in which the binding of ATP molecules determines the enzyme conformation. Such compounds and atoms were referred to as transplants. The proposed algorithm allows for the transfer of necessary structures into AlphaFold models in the cases where their direct analogues are found in the PDB database.
According to the statistical processing performed in the cited study[116], among the most frequently encountered transplants, one can highlight nucleotides (ATP, adenosine diphosphate, adenosine monophosphate, guanosine diphosphate, guanosine triphosphate, uridine-5'-diphosphate), cofactors [coenzyme-A, flavin adenine dinucleotide, flavin mononucleotide, glutathione, heme, nicotinamide adenine dinucleotide (NAD), pyridoxal phosphate, etc.], and metal ions (Ca2+, K+, Mg2+, Na+, Zn2+).
In the first stage, a search was conducted for template threedimensional protein structures meeting two criteria: homology of at least 25% and at least 85 aligned residues (using the BLAST algorithm in the LAHMA program). Information about cofactors was obtained from the CoFactor database[117]. A total of 2694 structures were transplanted, which accounted for 95% of all ligands (excluding xenobiotics) in the PDB database. Spatial alignment of complexes was performed using standard techniques according to the position of Cα atoms, with the quality of overlay being assessed by RMSD values. The protein atoms located within 6 Å of the cofactor position made up the transplantation area and were aligned separately. As a result, 586137 structurally modified AlphaFold models were obtained, with the total number of transplants exceeding 12 million. The initial validation of the AlphaFill algorithm was performed using proteins with 100% homology and the LEV (local environment validation) comparison function, which compares the cumulative RMSD of cofactors and the nearest amino acids in the obtained models with experimental data (Figure 5a). The results presented in Figure 5b indicate a relatively high correlation between LEV values and local RMSD. Overall, as expected, with increasing homology among amino acids that make up the pocket, the local RMSD decreases, despite a quite significant confidence interval (Figure 5c). Using scoring functions defined based on the overlap of van der Waals volumes of atoms for polyatomic molecules after transplantation (TCS is transplant clash score, Figure 4d), it was shown that TCS values correlate with LEV function values (Figure 5e)[116].
For the optimization of complex geometry with high overlap coefficients (Figure 5f), the authors[116] used the YASARA local minimization algorithm[118]. It is noted that after minimization of complexes with original TCS values close to zero, there is a slight increase in the overlap, characteristic of cases where the cofactor does not have nearby protein atoms after transplantation. Under the action of the force field, the site ‘compresses,’ which, considering the intermolecular components of the field, leads to partial overlap of atom volumes. In other cases, the minimization procedure significantly lowered the TCS values. An example of optimization using the AlphaFill algorithm for myoglobin is shown in Figure 6. Besides myoglobin, examples of transplants for zinc-dependent sites and kinase enzymes were discussed in the same paper[116]. Although the authors did not provide examples of algorithm validation in the context of docking of small molecules, it can be expected that in some cases, especially when the ligand affinity is, among other factors, determined by interaction with a cofactor, docking results will improve compared to the original model.
Liang et al.[119] thoroughly investigated the role of JMJD8 as an oncogene, which belongs to the JMJD protein family containing the Jumonji C (JmjC) domain in its structure. Proteins of this family can catalyze histone demethylation, similarly to HDAC (histone deacetylase-3) (deacetylation) and KDM (lysine-specific demethylase) (demethylation). However, such enzymatic activity was not demonstrated for JMJD8, likely due to mutations in the JmjC domain, while the N-terminal domain provides JMJD8 localization in the endoplasmic reticulum, indicating its role in folding. Besides, JMJD8 directly interacts with partner proteins, such as PKM2, leading to accelerated glycolysis. Specifically, it was found that the expression of this gene correlates with immunosuppression, DNA repair, and the activity of the CD276 protein, which is involved in regulating the T-lymphocyte immune response. Although the authors did not detect demethylase activity of JMJD8 in any of the conducted tests, they observed an association with several methyltransferases of other types.
Analysis of the gene expression profile using the cMap program[120], particularly for the JMJD8 gene, in various tumour cells identified 26 molecules the impact of which induced statistically significant changes in the expression of this gene. Among these, 6 molecules were categorized as HDAC inhibitors. In this context, Liang et al.[119] used the AlphaFold JMJD8 model for docking of four molecules [XMD-1150 (5), XMD-892 (6), genipin (7), and THM-I-94 (8), Figure 7a] to predict their potential direct binding to JMJD8. The docking procedure, identification of the potential binding site, and preprocessing were carried out using the Discovery Studio v4.5 program (LibDock module).k The modelling results (Figure 7b) showed that XMD-1150 and XMD-892 are incapable of interacting with the protein, while genipin and THM-I-94 demonstrated relatively high scoring functions (LibDockScore: 104.95 and 131.25, respectively).
Since the cited paper[119] does not provide detailed information about the specific features of the computer experiment and lacks experimental validation of the JMJD8-targeted mechanism of action for the selected molecules, assessing the role of the AlphaFold model in this work is quite challenging. It is worth noting that THM-I-94 contains a hydroxamic acid fragment typical of HDAC inhibitors, which interacts with the Zn2+ cation in the HDAC binding site, and the JMJD8 binding site visually resembles the catalytic site in the HDAC structure. This indirectly suggests the possibility that the molecule interacts with the studied protein. However, since, as mentioned earlier, the original AlphaFold models do not have cofactors in their structure, the docking results can be questioned if the authors did not perform a proper preprocessing procedure, which is not not mentioned in the cited publication[119].
To discover new selective inhibitors of the enzyme OfHex1 (O. furnacalis), which participates in the hydrolysis of terminal N-acetyl-D-hexosamine residues in N-acetyl-β-Dhexosaminides, as insecticides, Satti et al.[121] described an approach based on the AlphaFold model. This work is related to agrochemistry, but the approaches to studying the mechanism of action and initial optimization of the structure of primary hit molecules are similar to those used in medicinal chemistry. In the first stage, to obtain comparative characteristics, the authors used available information for the specified enzyme from three organisms — O. furnacalis, Homo sapiens, and T. pretiosum. For enzymes from the first two organisms, the required crystallographic data were found in the PDB database: 3NSN (2.10 Å) and 1NP0 (2.5 Å). According to analysis, the overall amino acid homology was 40.11%, and the all-atom RMSD = 1.21 Å. In the case of T. pretiosum, an AlphaFold model was used (pLDDT = 86.75), with the Molprobity score[122] being 1.61 in the 92nd percentile (N = 27675, 0–99 Å) and the volume overlap coefficient being 1.75 in the 99th percentile (N = 1784, all resolutions). The homology with the nearest experimental complex (5Y1B) is characterized by corresponding values of 36.41% and 0.75 Å. Then, crystal preparation was carried out using the Maestro program, in particular, water molecules more than 5 Å away from the protein and ligand atoms were excluded from consideration, hydrogen atoms were added, and missing chain sections were built in the PRIME module. Complex minimization was performed using the OPLS4 force field.
Subsequently, the authors[121] compiled a library of commercially available molecules containing moieties typical of insecticides. In total, more than 20000 molecules were selected, which underwent standard preprocessing using the LigPrep module. For each structure, no more than 32 starting three-dimensional representations were generated for docking (totaling 44943 conformations). To validate the docking models, the authors predicted poses for ligands from the aforementioned crystals [TMG-chitotriomycin (9) and NAG-thiazoline (10) for 3NSN and 1NP0, respectively]. It was shown that using the constructed models, the true poses of the mentioned ligands are reproduced (Glide score = −12.95 and −6.53 kcal mol–1 for O. furnacalis and Homo sapiens, respectively.) In the AlphaFold model for a berberine derivative (11), a Glide score of –6.12 kcal mol–1 was obtained (T. pretiosum) (Figure 8a). The predicted binding mechanisms of the mentioned ligands are presented in Figure 8b. For docking of experimental structures of potential ligands, the Glide module was used (XP mode, that is, extra precision), and for the three poses with the best Glide scores, the free binding energy was evaluated using the MMGBSA method (molecular mechanics with generalized Born and surface area)[123]. The MD method in the Desmond module (Schrödinger LLC) was then applied to refine the binding mechanism and optimize the potential energy (using the OPLS4 force field), with the TIP3P scheme being used for water molecules, and system neutralization was achieved using Na+ ions. Details of this experiment can be found in the original publication[121]. Ultimately, for each conformation, the 5 most probable MD results were selected. According to the modelling data (see Figure 8a), the active conformations for molecules 9 and 10 correspond to experimental data, while for compound 11 significant differences are observed, despite a high degree of spatial homology for the pockets.
As a result of docking of selected structures of potential ligands, 15 compounds (18 conformers) were identified, which are predicted to bind better than the control molecule 9 exclusively to OfHex1 (Glide score ≤ –12.95 kcal mol–1). Examples of molecules with the best Glide scores (12–15) are presented in Figure 9.
The optimal poses for all 18 conformers were analyzed using the MD method in two stages (5 and 40 ns). After the first stage, 5 molecules were selected for which the RMSD over the trajectory did not exceed 3 Å. After the second stage, the greatest stability of complexes was predicted for three molecules — 9, 13, and 15. The authors[121] did not provide the results of biological testing for the selected compounds, which does not allow for an assessment of the efficiency of their approach. Furthermore, no attention was given to the AlphaFold model, and possible reasons for the discrepancy in active conformations in the case of T. pretiosum were not explained.
Lokhande et al.[124] used several AlphaFold models of viral proteins such as thymidylate kinase (A48R), DNA ligase (A50R), scaffolding protein D13 (D13L), palmitoylated EEV membrane protein (F13L), and bovine cysteine protease (I7L), to identify potentially active molecules against monkeypox virus (MPXV), which belongs to the Orthopoxvirus genus of the Poxviridae family. Recent studies have identified a significant homology (96.3%) between MPXV and the smallpox virus[125]. Therefore, the authors[124] selected the mentioned targets, for each of which, except A48R, known ligands exist: tecovirimat (ST-246) for F13L, TTP-6171 for I7L, rifampicin for D13L, and mitoxantrone for A50R. Preprocessing of the selected AlphaFold models was carried out using the Maestro program, following the standard protocol in the Protein preparation module. The SAVES (v6.0) program was used to construct Ramachandran plots, and the verification and analysis of three-dimensional structures were conducted in the ERRATl and ProSA programs[126]. The molecules for docking were selected from the ChemDiv collection, PubChem, and DrugBank databases. A total of more than 206000 structures were selected, which, after filtering procedures [REOS (rapid elimination of swill) and PAINS (pan-assay interference compounds) filters], were reduced to 171000. Preprocessing and generation of starting three-dimensional representations for the final set of structures were performed in the LigPrep module (standard settings, OPLS4 force field).
As a result, more than 349000 three-dimensional structures were obtained, the affinity of which was assessed based on the docking results. The binding sites for reference molecules were determined by analysis described by Lam et al.[127] and the results from the SiteMap module in the Schrödinger program (Figure 10). To optimize the time and computational costs, the first stage of modelling was conducted in the high-throughput virtual screening mode and was followed by the application of the SP (standard precision) docking protocol for the top 10% of most promising compounds. In the final stage, for 10% of the most favourable conformations identified during the second stage, affinity was predicted in the XP mode. The likelihood of binding was assessed based on the Glide score. The stability of the predicted complexes and the affinity of ligands were analyzed using the MD method in the Desmond module, similar to what was done in the study cited above[121] (force field, OPLS 2005, cubic lattice 10 Å, 100 ns, TIP3P protocol, Nose-Hoover thermostat model, Martyna–Tobias–Klein barostat with isotropic coupling). Structural changes in the system were monitored based on RMSD and root-mean-square fluctuations of this parameter. Principal component analysis was used to visualize dynamic changes in the system. Complex energies were evaluated based on MM-GBSA calculations; the potential for interaction of molecules (virtual hits) with the binding site was assessed using calculations of the energies of the highest occupied and lowest unoccupied molecular orbitals, conducted using B3LYP and LDF functions[128] in the DMol3 module of the Discovery Studio program. The contribution of dispersion forces was calculated using DFT-D (dispersion-corrected density functional theory). AlphaFold models and corresponding pockets are presented in Figure 10. In particular, the authors[124] noted that the results published by Lam’s research group[127], correspond to positions predicted in the SiteMap module. The main characteristics of the sites are presented in Table 5.
Based on the constructed Ramachandran plots, it was established that no more than 0.6% of amino acid residues (approximately 3 amino acids) in each model do not correspond to experimental data (except for I7L), with ERRAT scores [ERRAT is the cost function for non-bonded interactions between atoms (C, N, and O) in proteins] being 97.95, 91.47, 85.31, 95.04, and 87.50% for A48R, A50R, D13L, F13L, and I7L, respectively. Docking results showed that reference molecules have higher Glide scores than the promising molecules (virtual hits) from the test sample (Figure 11). It is seen that for control molecules, Glide scores range from –4.92 to –4.52 kcal mol–1, while for test molecules the maximum value of this parameter was –7.83 kcal mol–1 for molecule (I7L), and the minimum value was –11.27 kcal mol–1 (I7L, molecule 36). MD results showed that the predicted complexes for reference molecules (except for the A48R target, which used an apo form) and compounds 16–40 are relatively stable. The principal component analysis results for the obtained trajectories provided the conclusion[124] that the binding of the most promising ligands causes significant changes in the structure of the apo form of the studied proteins, which presumably prevents the formation of a stable active conformation. For all investigated potential ligands, the energy difference between the highest occupied and lowest unoccupied molecular orbitals ranged from 0.0385505 to 0.2725508 eV, which, according to the authors, is related to the ability of molecules to interact with the pocket. Similar results were obtained using the DFT-D method. Like in several other mentioned works, the cited study[124] does not provide biological testing results for the selected molecules.
Nussinov et al.[129, 130] noted that the efficiency of using AlphaFold models depends on the task at hand, and their potential in fields such as the development of new drug molecules and medicinal chemistry is not fully clear for a number of reasons. Proteins are not static objects, especially near active sites, as is the case for many enzymes. Solvent molecules play an important role in the interaction of ligands with binding sites. Even single substitutions among the amino acids lining the pocket can have a significant impact on the affinity of small molecules. The authors emphasize that many enzymes exist in various states, citing kinases and 5-HT5A serotonin receptors as examples.
The reasons listed above, among other factors, do not allow AlphaFold structures to be considered as suitable models for conducting standard computer modelling procedures without additional modification. Researchers compare AlphaFold models to photographs, not to the living systems that proteins are complex dynamic entities whose degrees of mobility are far from being limited to two states (active and inactive). Identifying allosteric binding sites using AlphaFold models is likely the most challenging task, as allosteric sites may form only in certain states of the protein, for example, during interaction with a partner molecule, or depend on surrounding conditions, particularly pH. An example is the development of the allosteric inhibitor asciminib against the BCR-ABL1 oncoprotein for chronic myeloid leukemia[131]. However, ligand binding to an allosteric pocket does not always guarantee a clear effect due to poorly predictable conformational transitions. For instance, inhibitors of integrins aIIbb3 and a4b1 stabilize the active conformation, demonstrating properties of partial agonists, which posed a serious problem during clinical trials[132].
Considering the above, as one of the possible solutions to the listed problems, Nussinov et al.[129, 130] suggested integrating information about functionally significant states of the protein into AlphaFold models, for example, about the kinase DFG-in/ DFG-out conformation, using pharmacophores for the inactive, typically more stable state of the protein, which in most cases is realized in AlphaFold models. The authors specifically mentioned a publication[133] discussing an approach that takes account of various states of GPCR proteins in the AlphaFold algorithm.
4.2. Design of novel small molecules
Recently, Ren et al.[134] published the results of a study in which they managed to identify the protein CDK20 as a new potential target for the drug therapy of hepatocellular carcinoma using the PandaOmics program[135]. In the absence of experimental data on the structure of the selected target, the authors applied the AlphaFold algorithm to construct its three-dimensional model. Using the generative platform Chemistry42[136], a series of new stage, only 6 molecules were synthesized, two of which were active. Apparently, the introduction of a pyrazole moiety into the molecule significantly influenced the activity, which is not surprising considering the numerous examples in the development of kinase inhibitors, where such acceptor moieties interacted with the lysine (in this case, Lys88) amino group either directly or through bridging water molecules. In the test using the Huh7 cell line expressing CDK20, compound 42 demonstrated relatively high activity (IC50 = 208.7±3.3 nM), while the activity against HEK293 was 1706.7±670.0 nM (IC50, selectivity index SI = 8.2). The authors noted that the constructed three-dimensional model (AF-Q8IZL9-F1-model_v1) did not allow for a correct docking procedure and was manually modified, for example, the C-terminal section (Pro303–Gly346) was removed. For modelling, from one to 302 amino acids were used, while the model corresponded to the DFG-in conformation, and the charged amino acids Asp87 and Glu90 were located in an area accessible to the solvent.
Ren et al.[134] were the first to demonstrate the effectiveness of using AlphaFold to create new active ligands against a kinase not previously described as a potential target for the therapy of hepatocellular carcinoma. It is specified that the new target is considered to be a protein that is ‘targetable’ (without explaining the term), has the status of ‘new target’ in the PandaOmics program, has not been addressed in any clinical trials in the last three years, and is not a target for known approved drug molecules. This definition, in our opinion, does not fully correspond to the concept of a ‘new target.’ The term ‘targetable’ is interpreted differently by medicinal chemists, cheminformaticians, and clinicians. For example, from the perspective of clinical pharmacology, ‘targetability’ means that the protein and the drug molecule acting on it have proven themselves during clinical trials as an effective strategy in treating a specific disease with acceptable side effects and a suitable clinical outcome, which contradicts the authors’ definition. Moreover, the rationale behind the choice of a period during which no results of clinical research should be published (the last three years) is unclear. Likely, the authors[134] adhere to a definition appropriate in the field of medicinal chemistry, where ‘targetable’ means that examples of small molecules acting on the selected target are known, which de facto excludes the possibility of correctly applying the term ‘new target.’ Specifically, the cited paper[134] claims that molecule 42 contains a new fragment (based on the Tanimoto coefficient value) capable of binding to the hinge region, absent in known CDK20 inhibitors.
The metric mentioned for evaluating the novelty of structures is not typically used: novelty can only be assessed based on a thorough literature and patent search. The authors[134] have filed the patent application WO2023138412 (A1); however, close analogues of the presented structures were previously described as, for example, ASK1 kinase inhibitors (compounds 43 and 44, see Figure 12)[137]. Essentially, in the structure of ISM042-2-048, there was an isosteric replacement of the phenyl group with a pyrrole ring while maintaining the inverted amide group while the pyrazole moiety, which in molecule 43 also contacts the lysine residue similarly to Lys88, was moved to the 7-position of the benzopyrimidine. However, docking results for ISM042-2-048 show the pyrrole proton interacting with the carbonyl group of the peptide backbone of Ile10 through the formation of a hydrogen bond, absent in the case of the phenyl group. Given the above, it could be assumed that testing molecule 43 against CDK20 or compound 42 against ASK1 would provide a better evaluation of the uniqueness of the approach described by Ren et al.[134]
It should be noted that among the published crystallographic data, we discovered a co-crystal of the topological analogue 45 (AZD-5438, a non-selective inhibitor of CDK kinases)[138] in complex with CDK2 kinase (PDB: 6GUH, crystal resolution 1.50 Å). Alignment of 6GUH and AF-Q8IZL9-F1-model_v1 showed that the pockets of CDK20 and CDK2 have high homology (Figure 13). In the case of AZD-5438, the imidazole acceptor nitrogen provides interaction with the lysine residue through a water molecule. Given that Ren et al.[134] did not present selectivity research results for ISM042-2-048, at least regarding the CDK kinase family, it can be speculated that comparable results could have been achieved using a simple homologous model. Despite the mentioned observations, in the studied research, the AlphaFold model allowed medicinal chemists, albeit not without expert modification of the modeled CDK20 structure, to develop hit molecules with inhibitory activity against CDK20 kinase.
Another study[139] reported by the same research group addresses the development of selective SIK2 kinase inhibitors using the AlphaFold model (selective SIK2 inhibitors are described in the literature, for example, ARN-3236, Ref.[140]). Specifically, the authors compared their own homologous model, constructed based on the previously proposed binding mechanism of the non-selective SIK inhibitor MRIA9[141], using the AlphaFold model, while details of the reproduction of the homologous model are not provided. It is noted that when overlaying the two models, significant differences (7.58 Å) are observed in the P-loop (Asn30) in the binding sites (Figure 14). We reproduced the homologous model in accordance with the methodology described by Tesch et al.[141], using the complex structure 7B30 as a template and the SWISS-MODEL program, and confirmed these differences.
Zhu et al.[139] performed docking simulations for four known ATP-competitive inhibitors with both the constructed homologous model and the model obtained from AlphaFold (AF-Q9H0K1-F1-model). It was indicated that the selected compounds interact with the hinge region; however, in the case of GLPG-3970 (46), the 3,4-dihydroisoquinolin-1(2H)-one moiety is located at the exit of the pocket (Figure 14b), unlike the docking results obtained using the AlphaFold model, where this moiety is located near the gatekeeper area, and the methoxy group occupies a pocket formed by amino acids in this region (Figure 14c).
We attempted to reproduce the reported results and conducted docking simulations of GLPG-3970 with both models. The constructed homologous model was not minimized and only underwent standard preprocessing, as was the case with the AlphaFold model. We precisely replicated the binding pose of G-5555 in the SIK2 kinase binding site, but for GLPG-3970 in the case of the homologous model, we did not observe the differences indicated by Zhu et al[139]. Key contacts with the hinge region are maintained, and the oxygen of the carbonyl group interacts with the lysine residue (Lys49). It is important to note that we obtained a similar pose for the hit molecule 46 described previously[139] (Figure 14d). Conversely, poses where the dihydroisoquinoline moiety is located at the exit of the pocket were obtained precisely during docking simulations with the AlphaFold structure. It should be noted that based on MD results, the authors considered the pose close to the one that was a priority in our computational experiment using the simple homologous model, but with a different torsion angle relative to the bond connecting the dihydroisoquinoline and thiazole moieties. The main focus in the previous publication[139] is the interaction of the methoxy group with the gatekeeper area, which, in particular, correlates with the results of structure–activity relationship analysis presented in that study. However, it remains unclear why the AlphaFold model better describes the patterns observed in the biological experiment. As a result, we were unable to confirm the significance of the AlphaFold model in this study. Nonetheless, using the Chemistry42 program, structures of new active compounds were generated, among which 47 and 48 demonstrated comparatively high activity in vitro: IC50 = 23 and 0.7 nM, respectively. In particular, high selectivity was noted for molecule 48 (SI = 24 and 200 for SIK2/SIK1 and SIK2/SIK3, respectively). At a concentration of 100 nM, no comparable activity was observed against other ten kinases, including AMPK kinase. The authors explained this by the presence of the Thr96 residue in the gatekeeper area of this kinase (unlike methionine in the SIK2 structure), affecting the binding of the methoxy group.
Mendes et al.[142] utilized the AlphaFold domain model of diacylglycerol kinase (DGK) and the molecular probe TH211 (49) (Figure 15), which is specific to tyrosine and lysine residues in the DGK active site (RF001-sites)[143], to identify potential binding areas for small organic molecules across a range of ten DGK isoforms. To study this, chimeric DGK family proteins were created, incorporating the C1 domain (tandem C1A and C1B domains), which is responsible for regulatory protein– protein interactions with partner proteins and provides specificity towards the substrate (diacylglycerol). Biological experiments demonstrated that TH211 blocks the catalytic activity of all isoforms through covalent interaction, with binding sites being identified not only in the C1 domain. Using AlphaFold models, the authors then mapped pockets by tyrosine and lysine residues in all isoforms (for tyrosine and lysine residues, pLDDT > 70), including chimeric proteins. As a result, clusters containing modified amino acids were identified, for example, for DGKα (K384, K543, Y544, K547), DGKζ (K502, K516, K521, K593; K311, K473), DGKγ (K356, Y358, Y535, K542), and DGKδ (K271, K198, K337). Additionally, the close spatial arrangement of the C1 and catalytic domain in the DGKα structure predicted by AlphaFold correlates with experimental data. This approach provides structural information about potential small molecule binding sites to DGK family proteins and can be used for developing selective inhibitors. AlphaFold models have also been used by other authors for predicting binding sites, for instance, in the structures of phosphatase PPM1D/Wip1[144], KRAS[145], andsacsin[146].
Li et al.[147], using AlphaFold models for the protein PcOSBP (P. capsici), developed a series of new inhibitors–structural analogues of oxathiapiprolin (50–53) (Figure 16), possessing fungicidal activity. As a control structure, the authors used crystallographic data (PDB: 1ZHY, 1.5–1.9 Å resolution) for the KES1 protein (Saccharomyces cerevisiae) from the aforementioned family with a low degree of homology (less than 30%) to PcOSBP in complex with ergosterol, cholesterol, and 7-, 20-, and 25-hydroxycholesterols. Sequence alignments were carried out using the MUSCLE Web program(https://www.ebi.ac.uk/Tools/msa/muscle/). Initially, an AlphaFold model was built for the control protein, resulting in a comparatively low average RMSD (0.54 Å), based on which the authors concluded that the algorithm is suitable for modelling the spatial structure of PcOSBP. Despite somewhat contentious reasoning, using the MD method, the authors optimized the AlphaFold model in complex with oxathiapiprolin to achieve a stable conformation. For docking the synthesized compounds (both isomers), the LeDock program[148] was used. For each structure, 100 docking attempts were made, and the affinity of the ligands was assessed based on the LeDock score and visual analysis of the predicted interaction. The docking results are presented in Figure 16.
It was shown that the binding mechanisms of the reference molecule and synthesized compounds coincide due to their high structural homology. Essentially, the authors[147] applied the scaffold hopping approach and modified the pyrazole moiety of oxathiapiprolin while preserving the key interaction with the lysine residue (Lys 703). The predicted binding energy values (ΔGPB) for R- and S-oxathiapiprolin were –24.73 and –24.59 kcal mol–1, respectively (the authors attributed the different values, in part, to the presence of a hydrogen bond with the Asn767 residue in the case of the R-isomer). Comparable docking results were obtained for compound 53: ΔGPB = –23.37 (R) and –22.32(S) kcal mol–1. Indeed, the R-isomer of oxathiapiprolin showed higher activity against P. capsici than the S-isomer[149] [EC50 = 0.17(R) and 0.66(S) μg L–1; EC50 is the half-maximal effective concentration], despite the relatively small difference in binding energies. The fungicidal activity of the synthesized molecules was investigated against P. capsici, Peronophthoralitchii (P. litchii), and P. infestans; at a concentration of 0.01 μg L–1, compound 51 demonstrated a weaker inhibitory effect than the control molecule: 28.15, 32.62, and 44.15% respectively. Compound 52 showed stronger activity at the same concentration, namely 94.93, 84.60, and 95.9%, comparable to the activity of oxathiapiprolin. Compound 53 more selectively inhibited the growth of the mentioned organisms at a similar concentration (99.19, 70.67, 87.11%), which was particularly associated with the ethyl group, which, according to docking results, could occupy the hydrophobic section of the binding site.
It should be noted that the authors[147] conducted field studies with compound 53, which demonstrated its high antifungal efficacy. This work relates to agrochemistry; however, the approaches to studying the mechanism of action and initial optimization of the structure of primary hit molecules are similar to those used in medicinal chemistry. In the described publication, the AlphaFold model allowed the authors to predict the possible binding mechanism of new molecules and analyze the structure–activity relationship. At the same time, the model was optimized using the MD method before the experiment, which essentially does not allow assessing its initial quality and efficiency for VS purposes. Likely, using a similar approach and a simple homologous model built for the target protein, similar results could have been achieved. The more so, because in the supplementary materials, the authors noted a high overall threedimensional homology both between the model and 1ZHY (RMSD = 0.54 Å) and in the pocket area (RMSD = 0.65 Å).
5. AlphaFold-latest: a new AlphaFold version
In late October 2023, there was an announcement about the successful work on a new version of the AlphaFold-latest program.n Specifically, validation results were presented for the module predicting the spatial position of ligands (small molecules) for various docking methods, including AutoDockVina, Gold, and DiffDock. It was shown that AlphaFold outperforms many docking modelling algorithms in prediction accuracy using the PoseBusters validation sample[150]. The test examples used by the authors were crystallographic complexes reported from May 2022 to January 2023, which were not included in the training set (a total of 8856 complexes after filtering procedures), while the model itself was trained on examples published before September 2019. The spatial overlay of pockets for RMSD calculation was done using protein atoms within 10 Å of ligand atoms. It was noted, in particular, that some problems with the correct placement of ligands in protein models were solved in the new version of the program using docking algorithms discussed in the literature[112, 151]. However, the authors did not present results of comparison with the most commonly used commercial programs Glide and MOE (Figure 17a). The results of predicting protein–protein interaction in AlphaFold-latest, particularly for antibody–antigen pairs compared to the previous version (AlphaFold-2.3), are shown in Figure 17b. The accuracy of reproducing positions of nucleic acids and their three-dimensional structures, as well as comparison results with other methods, are reflected in Figure 17c. It is evident that AlphaFold-latest outperforms RoseTTAFold2NA[152] in accuracy but is slightly inferior to Alchemy_RNA2 (under CASP-15 conditions)[153, 154]. The results of reproducing covalent modifications are shown in Figure 17d. Examples demonstrating that in some cases, the AlphaFold-latest algorithm can predict the true pose of a ligand with good accuracy, unlike docking algorithms, are given in Figure 18, and the results of validating AlphaFold-latest using various complexes are shown in Figure 19.
For predicting protein–protein interactions and ligand–protein complex structures, test examples were used that do not have direct analogues in the training data. In the case of protein–protein interactions, only those protein pairs were selected for which there were no training examples with a homology greater than 40% (for the pair). For ligand–protein complexes (small molecules and glycans), the same homology threshold (40%) was used, combined with a Tanimoto coefficient of structural similarity for the molecules not exceeding 0.5. However, information on the homology of pockets, which could significantly contribute to predicting the resulting poses of small molecules, was not provided.
For the second validation set, crystallographic data on complexes of proteins with ligands were used, excluding glycans, covalent ligands, ions, and other molecules not related to therapeutic agents. The experiment results are shown in Figure 20, indicating that the algorithm can predict the poses of ligands for various proteins with comparatively high accuracy. It is clear that, in the foreseeable future, results with biological validation of the described approaches will emerge; such information is necessary to assess the effectiveness of predicting the affinity of small drug molecules.
6. Conclusion
Considering numerous scientific publications in high-impact journals and the results of regular CASP competitions, the AlphaFold algorithm can be considered a leader in the field of modelling three-dimensional structures of protein molecules. This algorithm is crucial for solving many bioinformatics tasks, including analyzing enzymatic reaction mechanisms and selfregulation, profiling mutagenesis sites, modelling protein– protein interactions, and processing X-ray crystallography data. However, most authors of the publications reviewed above agree that without preliminary preparation, AlphaFold models are limitedly suitable for medicinal chemists, especially for docking within typical VS. For example, based on the analysis of experimental results on reproducing true ligand poses[98], it was concluded that AlphaFold models are mostly unsuitable for VS; it was also noted that due to the peculiarities of training, such models resemble apo-forms of proteins, unlike holo-variants, which showed better results during docking simulations.
Wong et al.[105] concluded that using unmodified AlphaFold models for high-throughput virtual screening is ineffective due to their low classifying ability. The models showed results comparable to those obtained with a random set of molecules at the biological screening stage. Scardino et al.[114] also pointed out the unsuitability of AlphaFold models for dockingsimulations. Better results were obtained using experimentally determined spatial structures of proteins, and this trend is characteristic of different docking methods. The Heckelmann’s research group116 concluded that AlphaFold models are not designed to predict the positions of molecules unrelated to the peptide backbone structure, including cofactors, non-protein coenzymes, and small molecules. Other studies reviewed in this overview demonstrated that even when the overall homology of the modelled site does not significantly differ from the experimentally established one, it is possible to obtain positions significantly different from the true ones. Clearly, depending on the conditions in protein molecules, atoms do not occupy static positions, and the conformation of the protein capable of binding a ligand is realized in a single or a limited number of cases, which, in particular, was noted by Nussinov et al.[129, 130], who compared AlphaFold models to photos.
Based on the above, it can be asserted that AlphaFold models predict the positions of the peptide backbone atoms and the overall packing with relatively high accuracy, including those for protein molecules for which no close homologues are available. The same cannot be said about the positions of the amino acid side chain atoms lining the pocket, and this fact is critical for modelling the interaction of the ligand with the protein target. Despite this, in experiments for predicting the possible binding site and its geometry, AlphaFold models show better results than homologous models[151]. However, replacing just one amino acid in the binding site or incorrectly modelled positioning of its atoms can lead to a complete loss of ligand affinity. In some cases, irregular protein fragments are located in the binding sites of AlphaFold models, which almost entirely preclude the possibility of obtaining adequate docking simulation results. It must be noted that many publications do not provide results of biological testing of selected molecules, which does not allow for a full assessment of the applicability limits of such models in the early stages of new drug development.
Frequently, the use of standard homologous models for proteins with a high degree of homology in the binding site area leads to comparable and even better modelling results, as pointed out by Karelina et al[151]. Many researchers believe that the optimization of AlphaFold models in complex with ligands using molecular dynamics methods or in a flexible docking mode, where the positions of both ligand atoms and amino acids are refined in the force field gradient, can lead to computational models with higher predictive capability compared to unmodified AlphaFold models. However, such approaches require significant time and computational resources and also do not guarantee that the complex chosen as a result of modelling would adequately reflect the molecular mechanism of binding.
It is particularly important to note that for a medicinal chemist, the only and absolute measure of effectiveness for any computational approach is exclusively the results of biological validation. In this context, methods and algorithms are being developed that can automatically adapt AlphaFold structures for high-throughput docking. Specifically, we have proposed an algorithm called AlphaFoldOptimizer, based on machine learning methods, which allows predicting optimal positions of protein atoms in the binding site area and provides optimized three-dimensional models suitable for correct dockingsimulations (a corresponding publication is currently being prepared).
Despite the obvious drawbacks of AlphaFold models, there is no doubt about the rationale for using them in the development of new small medicinal molecules. Many researchers agree that the near future will see modified algorithms based on such models, for example, an improved version of AlphaFold (see Section 5), adapted for effective VS tasks, enriching the arsenal of the modern medicinal chemist with new useful tools.
7. List of abbreviations and designations
ABL1 — Abelson murine leukemia viral oncogene
homolog-1 (ABL1 tyrosine kinase),
ADRB2 — β2 adrenergic receptor,
AMPK — 5'-AMP-activated protein kinases (serine/threonine AMP-activated protein kinase),
ANDR — androgen receptor,
ATP — adenosine triphosphate,
auPRC — area under the precision–recall curve (precision and recall metric),
auROC — area under the ROC curve,
AziU3/U2 — aziridine synthase (Streptomyces sahachiroi),
BRAF — B-Raf proto-oncogene (serine/threonine kinase),
CASP — critical assessment of protein structure prediction,
CDK20 — cyclin-dependent kinase-20,
CDK2 — cyclin-dependent kinase-2,
CtaZ — GyrI-like protein polythioamide-binding protein,
CYPA — peptidyl-prolyl cis–trans isomerase A,
COX1 — cyclooxygenase-1,
DFT-D — dispersion-corrected density functional theory,
DFG — asp(D)-Phe(F)-Gly(G) (a conservative segment in the structure of kinase pockets),
DGK — diacylglycerol kinases,
DGPB — predicted binding energy,
DOOP — docking decoy-based optimized potential,
DRD3 — dopamine receptor D3,
EF — average enrichment factor,
EFS — evaluation function score,
EGFR — epidermal growth factor receptor,
ESR1 — estrogen receptor,
FABP4 — adipocyte fatty acid-binding protein-4,
FA7 — coagulation factor VII,
GdmN — carbamoyltransferase,
GPCR — G protein-coupled receptors,
HDAC — histone deacetylase-3,
HIF-1α — hypoxia-inducible factor 1α,
HSP90 — heat shock protein 90 homologue,
HXK4 — hexokinase-4,
IC50 — half-maximal inhibitory concentration,
IGF1R — insulin-like growth factor 1 receptor,
ITAL — integrin α-L,
JMJD8 — JmjC domain-containing protein 8,
KDM — lysine-specific demethylase,
KES1 — oxysterol-binding protein homologue-4,
KPCB — protein kinase C beta-type,
KITH — cytosolic thymidine kinase,
KRAS G12C — GTPase KRas (G12C mutation),
LBDD — ligand-based drug design,
LEV — local environment validation,
LFA1 — lymphocyte function-associated antigen-1,
LOMETS — Local meta-threading server,
MD — molecular dynamics,
MM-GBSA — molecular mechanics with generalized Born and surface area,
MOE — molecular operating environment,
MW — molecular weight,
NAD — nicotinamide adenine dinucleotide,
OfHex1 — chitinolytic β-N-acetyl-D-hexosaminidase,
PDB — protein data bank,
PDE5A — cGMP-specific 3’,5’-cyclic phosphodiesterase,
PcOSBP — oxysterol binding protein, PKM2–Pyruvate kinase type-2,
pLDDT — predicted local distance difference test,
PORCN-WNT3A — the complex of porcupine
O-acyltransferase and Wnt family member-3A,
PI5P4Kg — phosphatidylinositol 5-phosphate 4-kinase-g,
PRGR — progesterone receptor,
PTN1 — phosphatidylinositol-3,4,5-trisphosphate 3-phosphatase,
PYRD — mitochondrial dihydroorotate dehydrogenase,
pVHL — Von Hippel-Lindau disease tumour suppressor,
PNPH — purine nucleoside phosphorylase,
Q3 — a calculated parameter reflecting the accuracy of secondary protein structure prediction,
R2 — coefficient of determination,
RXRA — retinoic acid receptor-A,
SBDD — structure-based drug design,
SI — selectivity index,
SIK2 — salt inducible kinase-2,
UROK — urokinase-type plasminogen activator,
VS — virtual screening,
\( \tau \) and \( \tau_0 \) — intermediate values of torsion angles,
\( \phi \) and \( \psi \) — torsion angles.
References
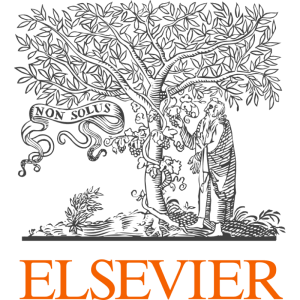
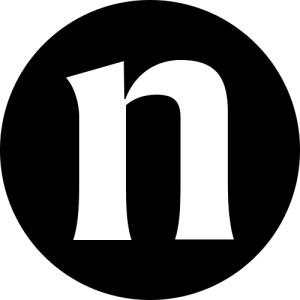
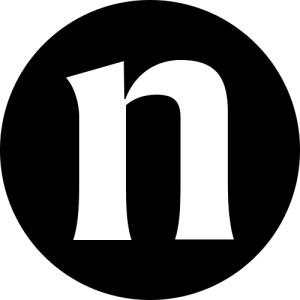
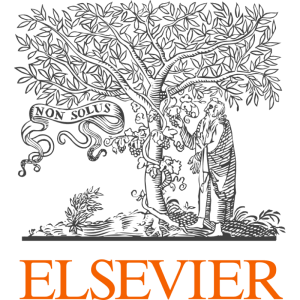
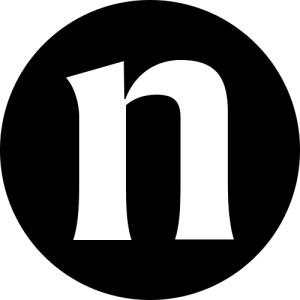
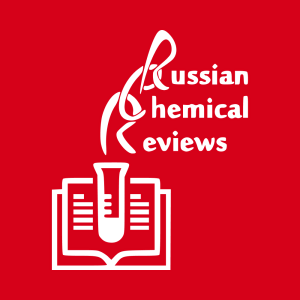
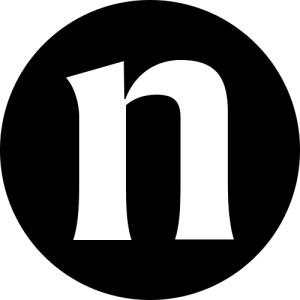
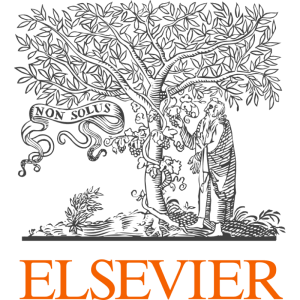
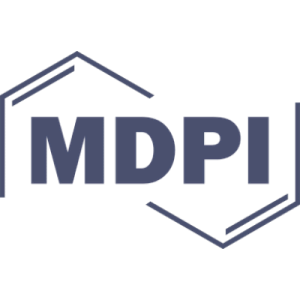
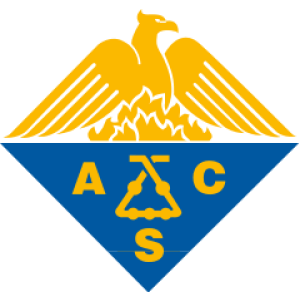
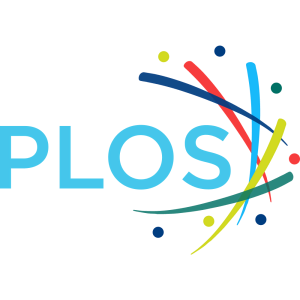
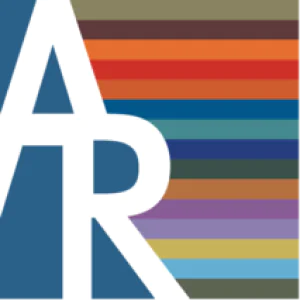
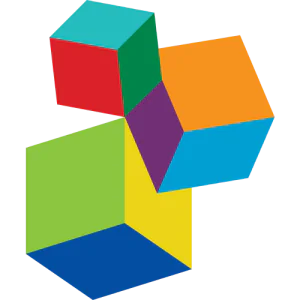
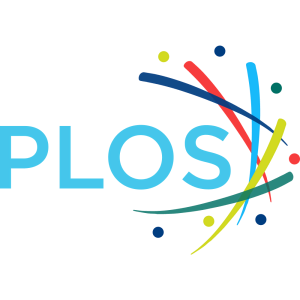
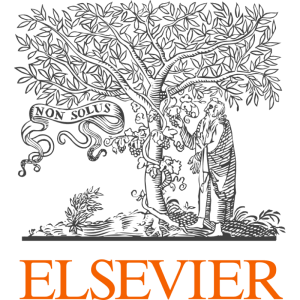
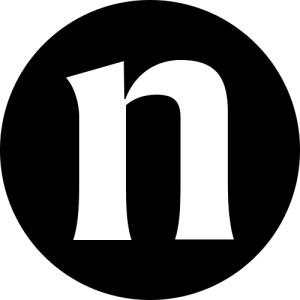
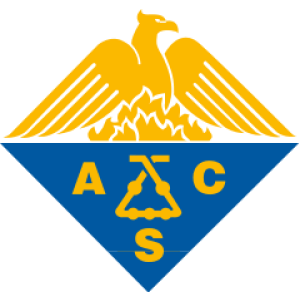
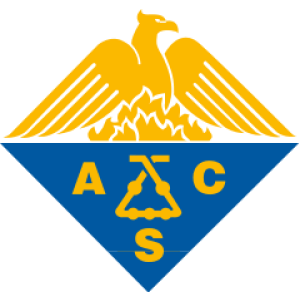
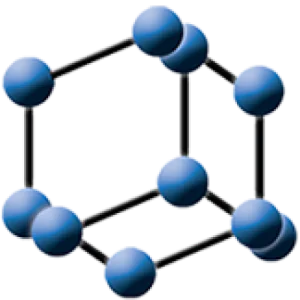
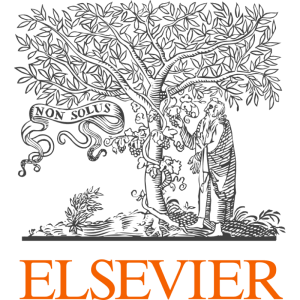
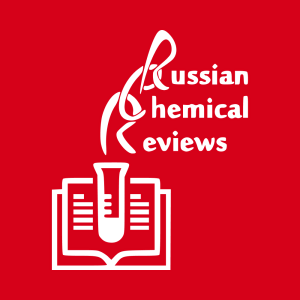
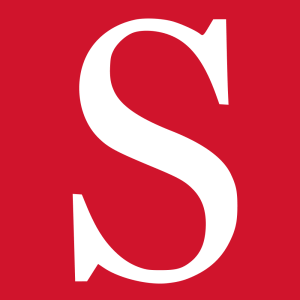
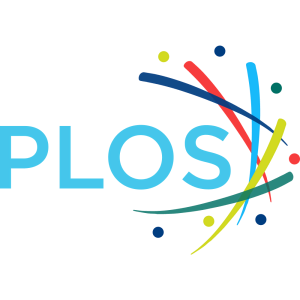
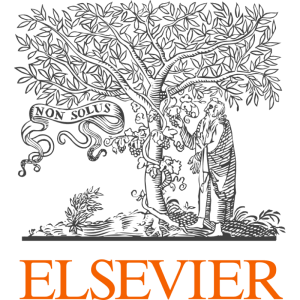
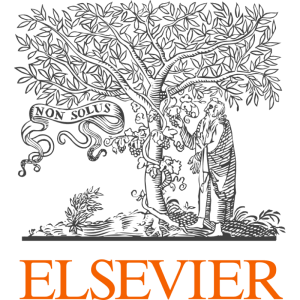
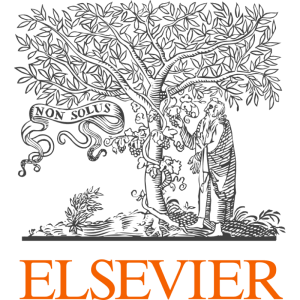
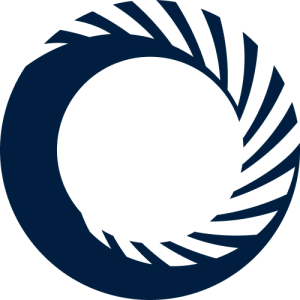
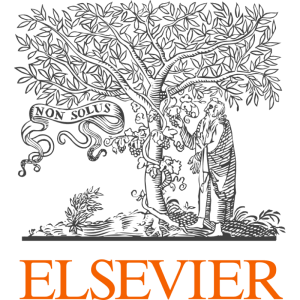
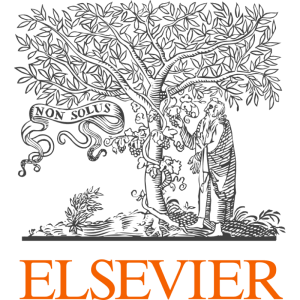
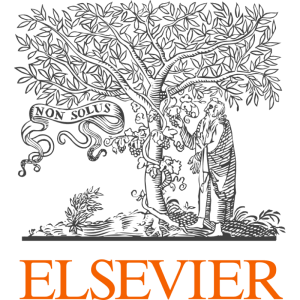
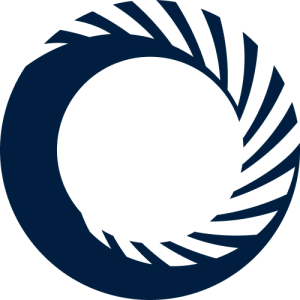
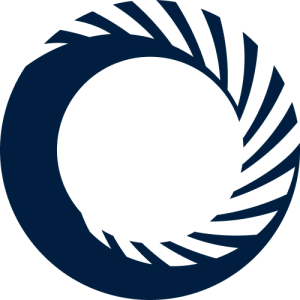
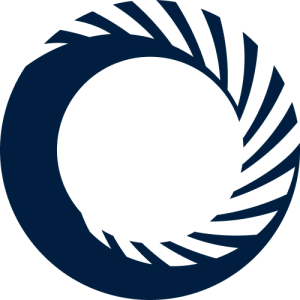
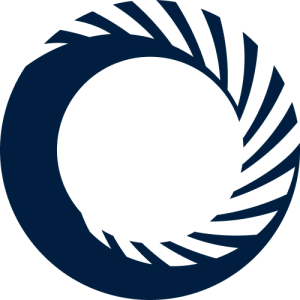
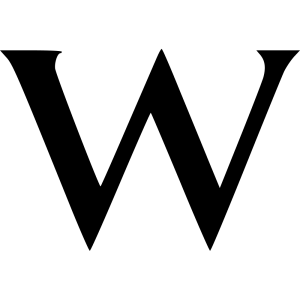
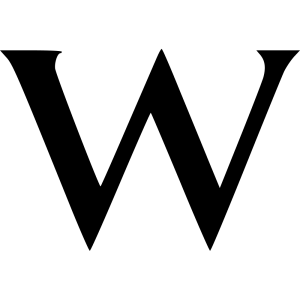
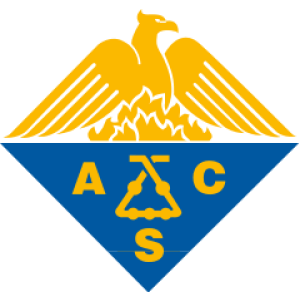
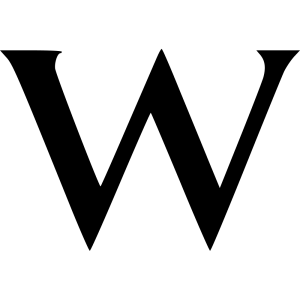
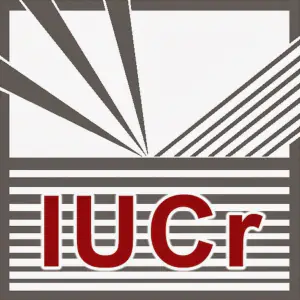
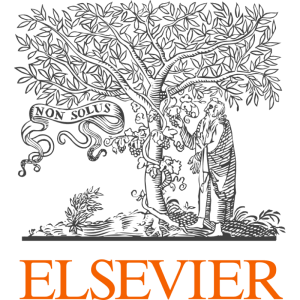
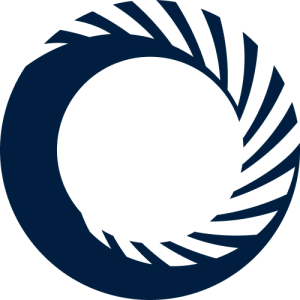
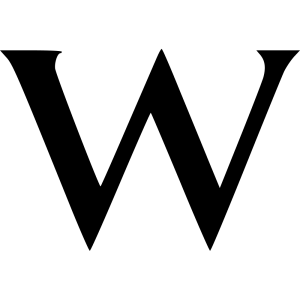
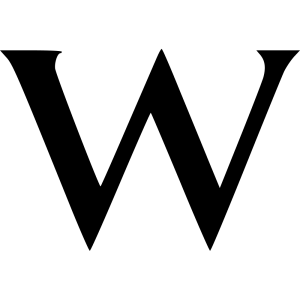
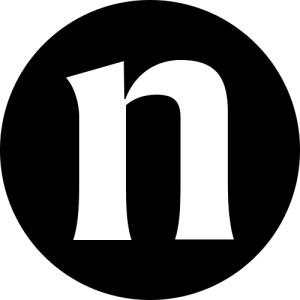
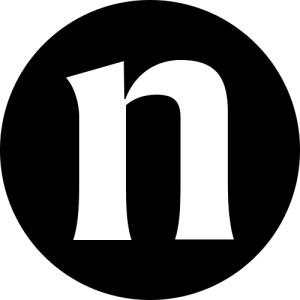
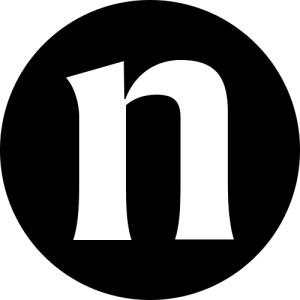
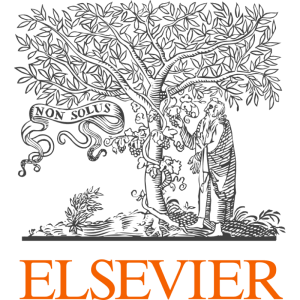
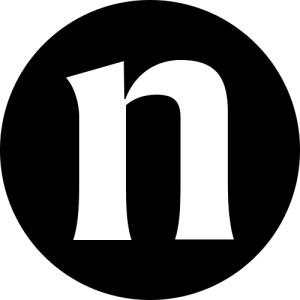
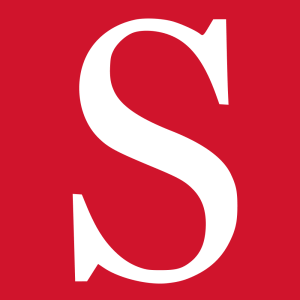
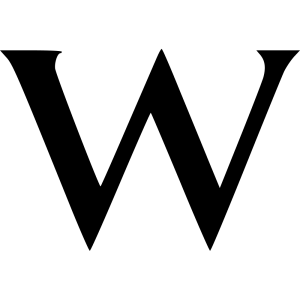
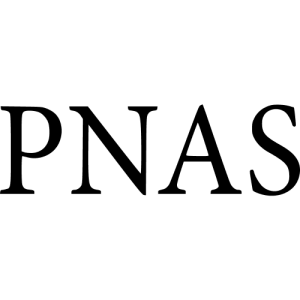
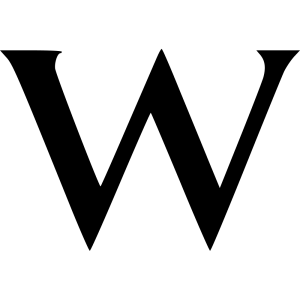
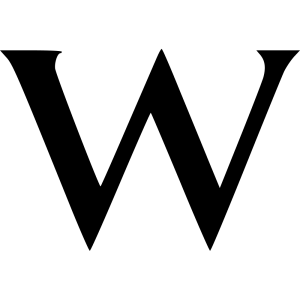
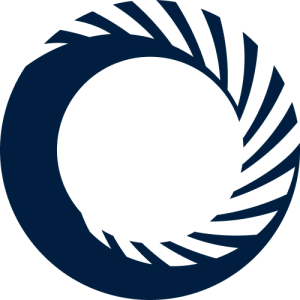
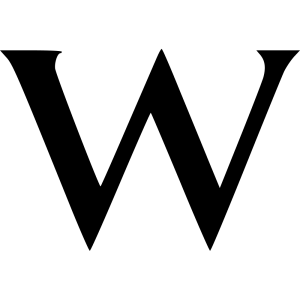
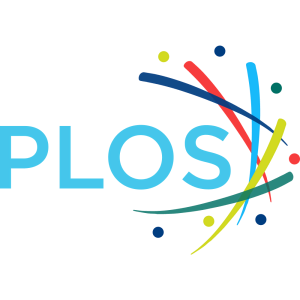
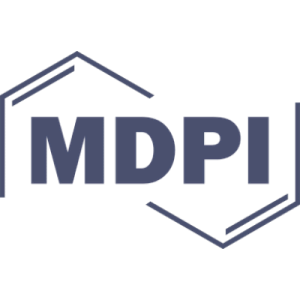
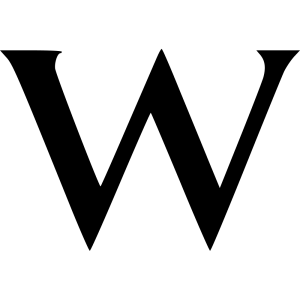
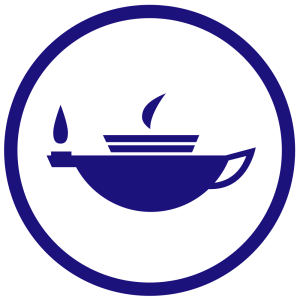
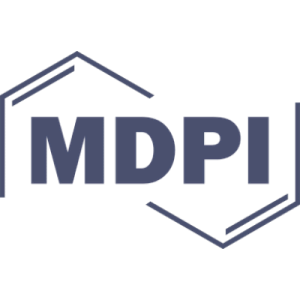
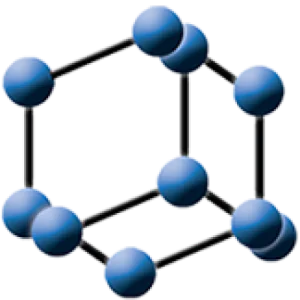
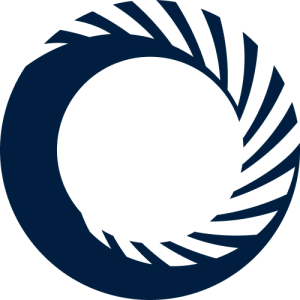
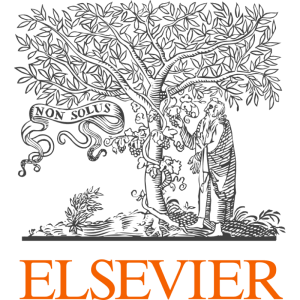
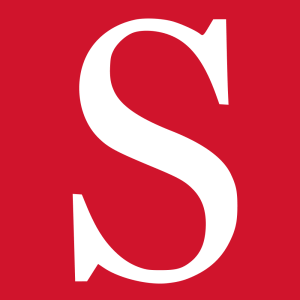
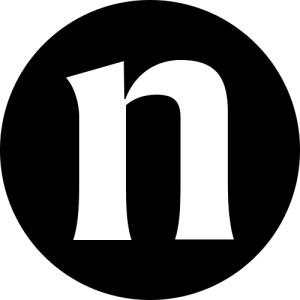
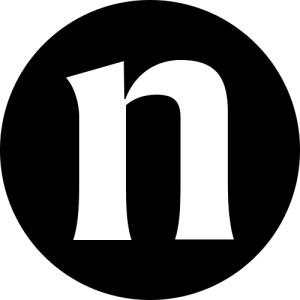
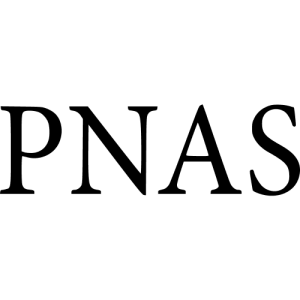
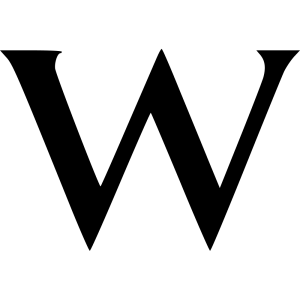
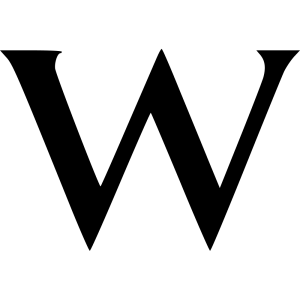
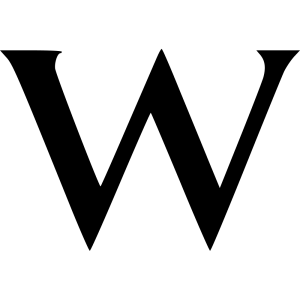
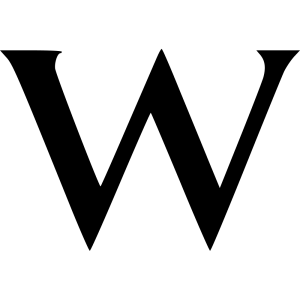
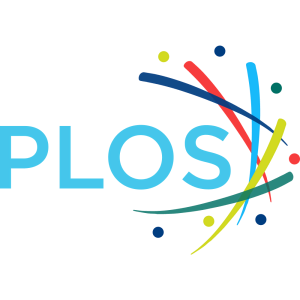
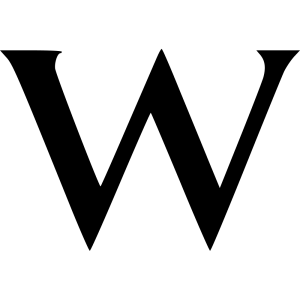
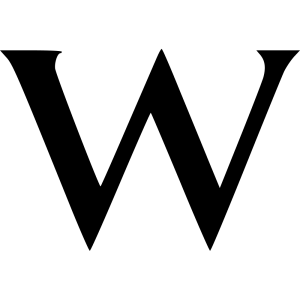
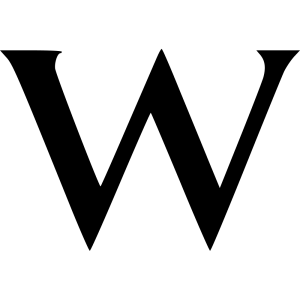
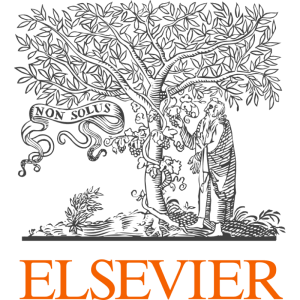
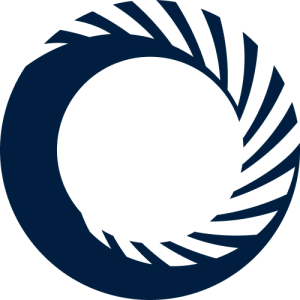
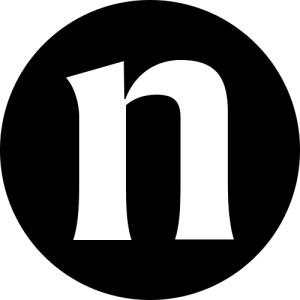
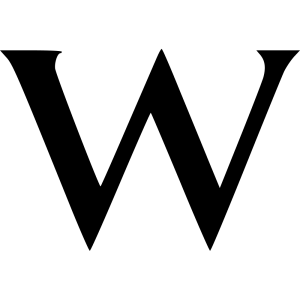
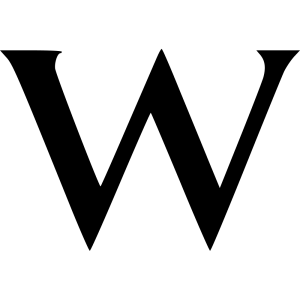
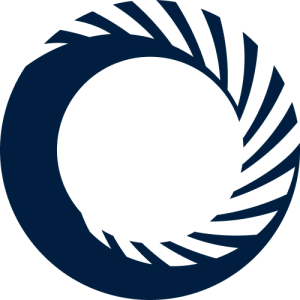
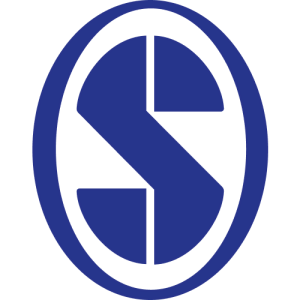
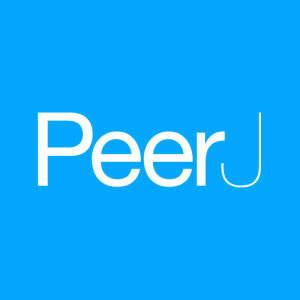
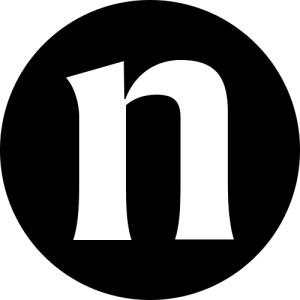
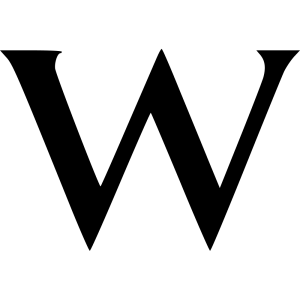
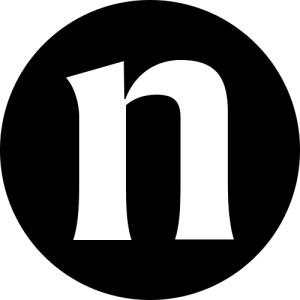
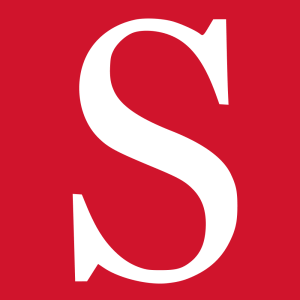
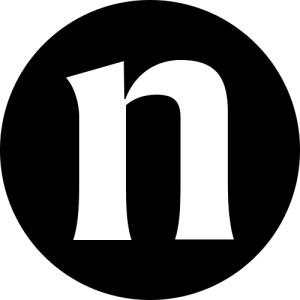
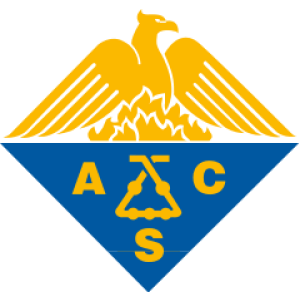
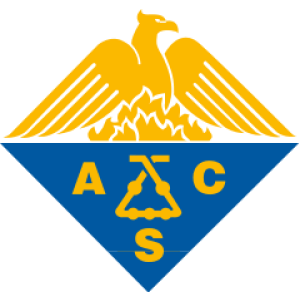
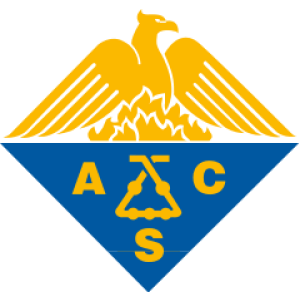
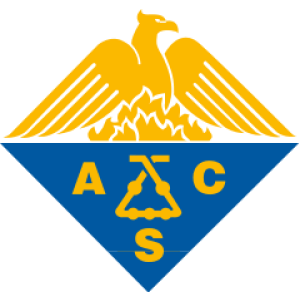
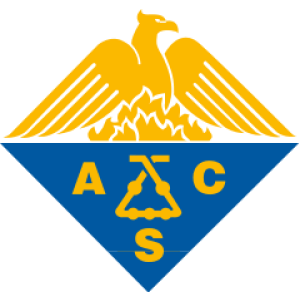
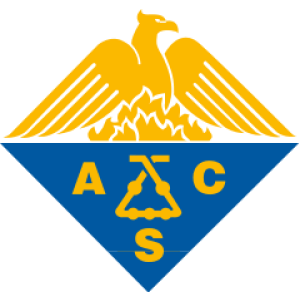
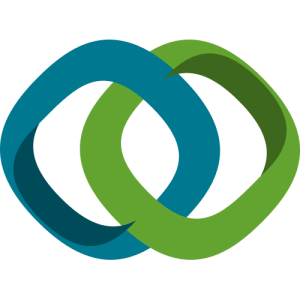
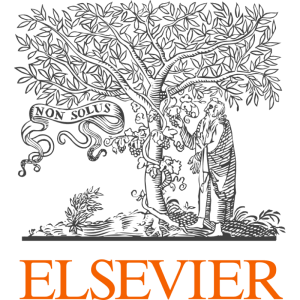
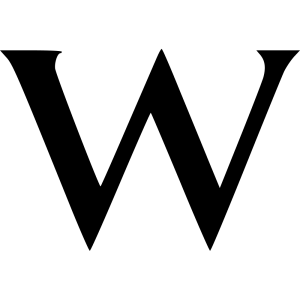
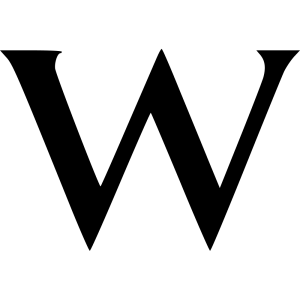
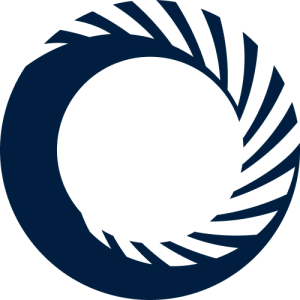
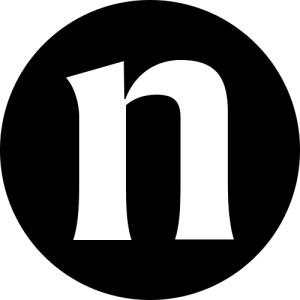
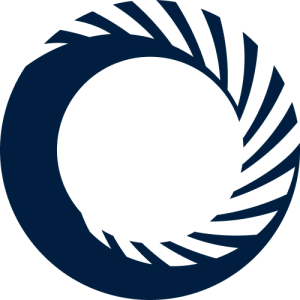
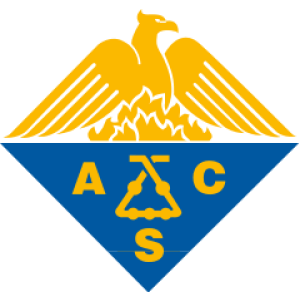
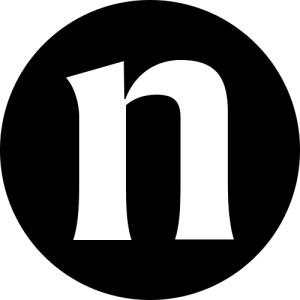
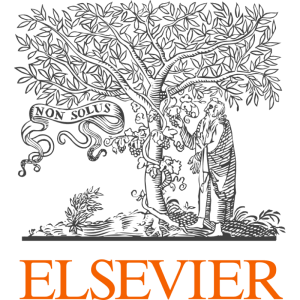
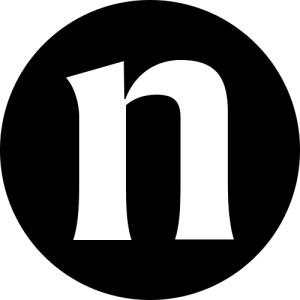
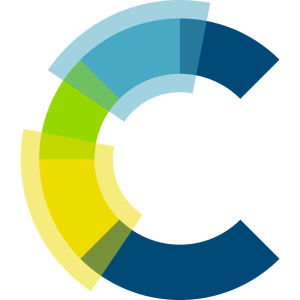
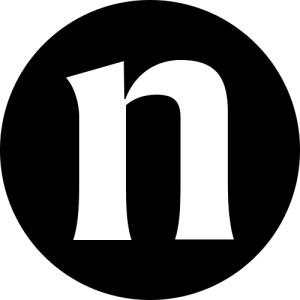
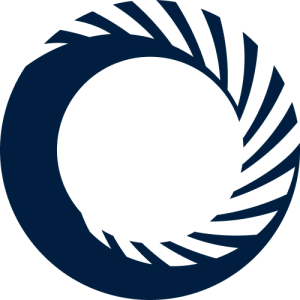
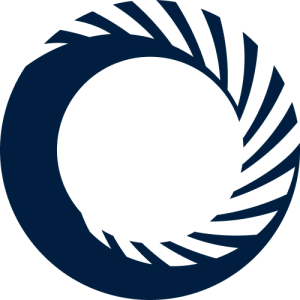
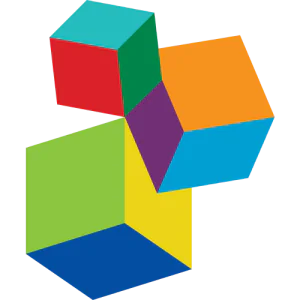
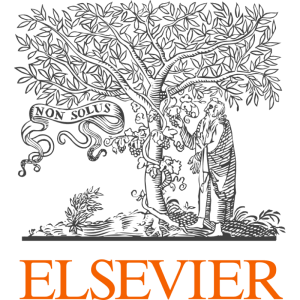
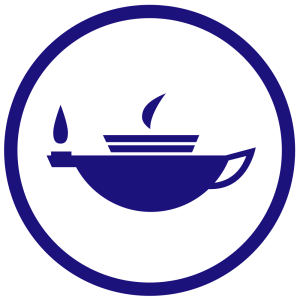
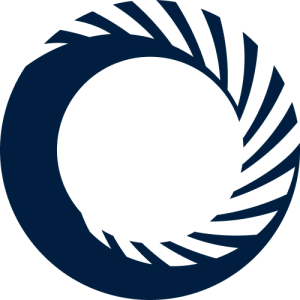
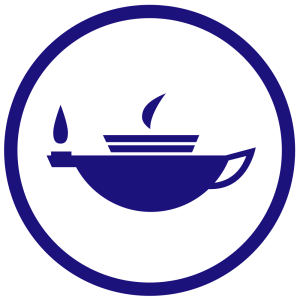
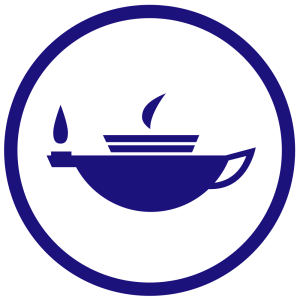
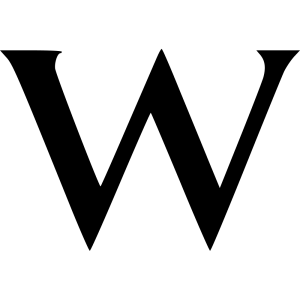
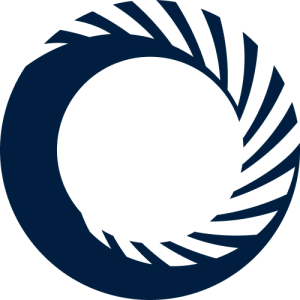
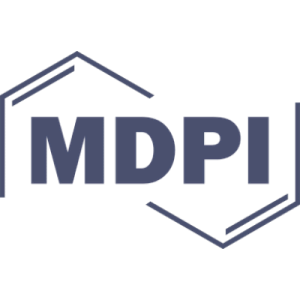
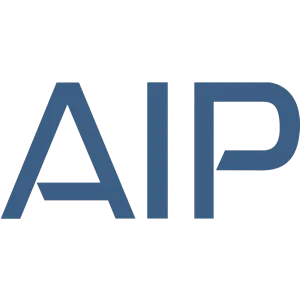
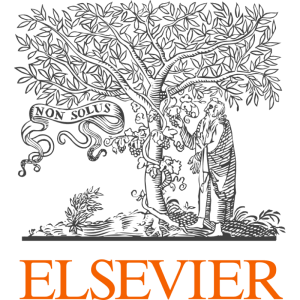
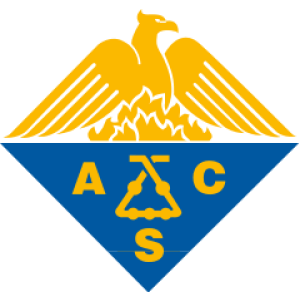
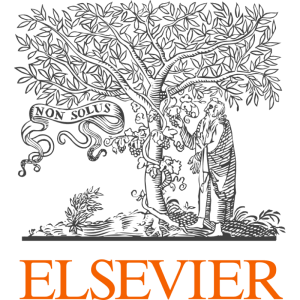
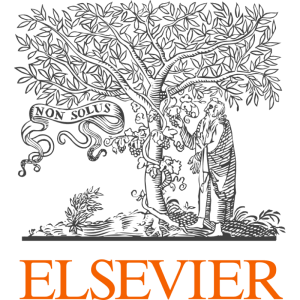
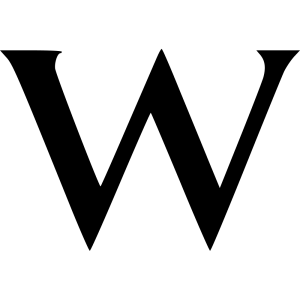
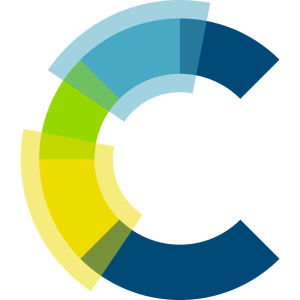
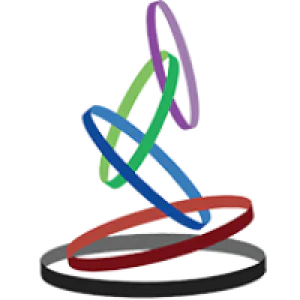
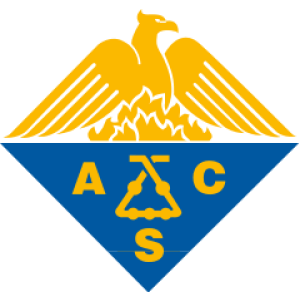
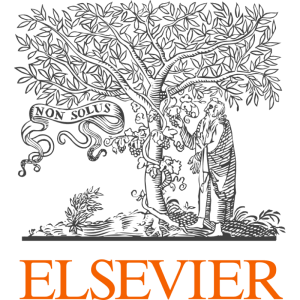
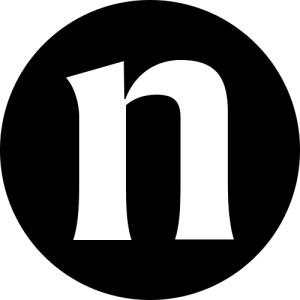
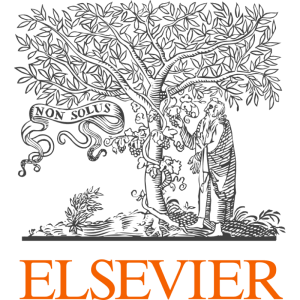
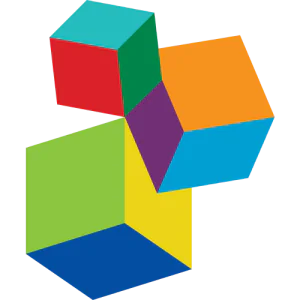
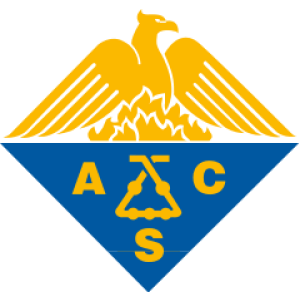
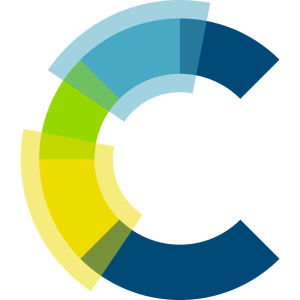
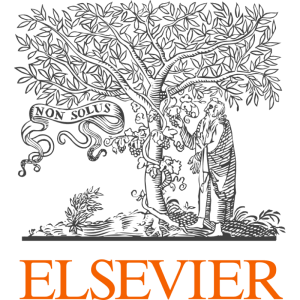
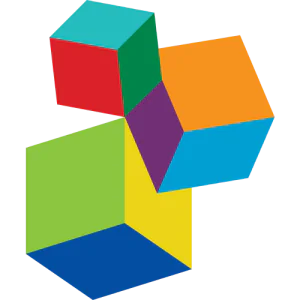
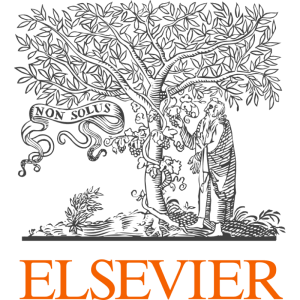
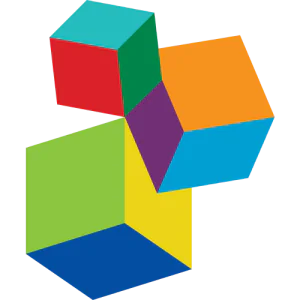
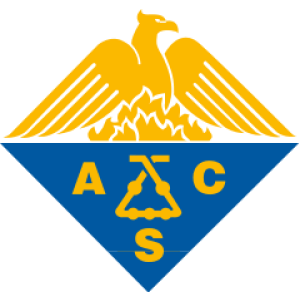
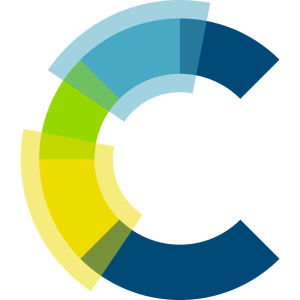
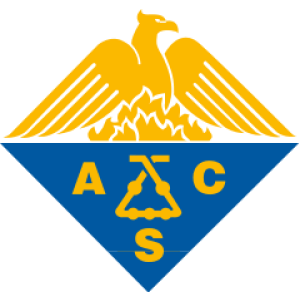
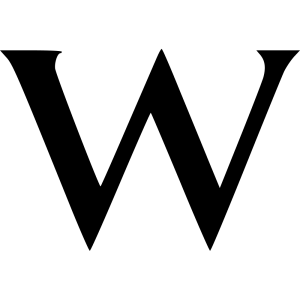
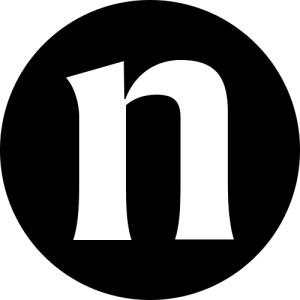